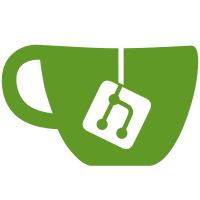
@ -0,0 +1,20 @@
|
||||
1. This question is validated if the output of the fitted model is: |
||||
|
||||
```python |
||||
LinearRegression(copy_X=True, fit_intercept=[[1], [2.1], [3]], n_jobs=None, |
||||
normalize=[[1], [2], [3]]) |
||||
``` |
||||
|
||||
2. This question is validated if the output is: |
||||
|
||||
```python |
||||
array([[3.96013289]]) |
||||
``` |
||||
|
||||
3. This question is validated if the output is: |
||||
|
||||
```output |
||||
Coefficients: [[0.99667774]] |
||||
Intercept: [-0.02657807] |
||||
Score: 0.9966777408637874 |
||||
``` |
@ -0,0 +1,13 @@
|
||||
# Exercise 1 Scikit-learn estimator |
||||
|
||||
The goal of this exercise is to learn to fit a Scikit-learn estimator and use it to predict. |
||||
|
||||
```console |
||||
X, y = [[1],[2.1],[3]], [[1],[2],[3]] |
||||
``` |
||||
|
||||
1. Fit a LinearRegression from Scikit-learn with X the features and y the target. |
||||
|
||||
2. Predict for `x_pred = [[4]]` |
||||
|
||||
3. Print the coefficients (`coefs_`) and the intercept (`intercept_`), the score (`score`) of the regression of X and y. |
@ -0,0 +1,25 @@
|
||||
1. This question is validated if the plot looks like: |
||||
|
||||
![alt text][q1] |
||||
|
||||
[q1]: ../images/w2_day1_ex2_q1.png "Scatter plot" |
||||
|
||||
2. This question is validated if the equation of the fitted line is: `y = 42.619430291366946 * x + 99.18581817296929` |
||||
|
||||
3. This question is validated if the plot looks like: |
||||
|
||||
![alt text][q3] |
||||
|
||||
[q3]: ../images/w2_day1_ex2_q3.png "Scatter plot + fitted line" |
||||
|
||||
4. This question is validated if the outputted prediction for the first 10 values are: |
||||
|
||||
```python |
||||
array([ 83.86186727, 140.80961751, 116.3333897 , 64.52998689, |
||||
61.34889539, 118.10301628, 57.5347917 , 117.44107847, |
||||
108.06237908, 85.90762675]) |
||||
``` |
||||
|
||||
5. This question is validated if the MSE returned is `114.17148616819485` |
||||
|
||||
6. This question is validated if the MSE returned is `2854.2871542048706` |
After Width: | Height: | Size: 39 KiB |
After Width: | Height: | Size: 53 KiB |
@ -0,0 +1,43 @@
|
||||
# Exercise 2 Linear regression in 1D |
||||
|
||||
The goal of this exercise is to understand how the linear regression works in one dimension. To do so, we will generate a data in one dimension. Using `make regression` from Scikit-learn, generate a data set with 100 observations: |
||||
|
||||
```python |
||||
X, y, coef = make_regression(n_samples=100, |
||||
n_features=1, |
||||
n_informative=1, |
||||
noise=10, |
||||
coef=True, |
||||
random_state=0, |
||||
bias=100.0) |
||||
``` |
||||
|
||||
1. Plot the data using matplotlib. The plot should look like this: |
||||
|
||||
![alt text][q1] |
||||
|
||||
[q1]: images/w2_day1_ex2_q1.png "Scatter plot" |
||||
|
||||
2. Fit a LinearRegression from Scikit-learn on the generated data and give the equation of the fitted line. The expected output is: `y = coef * x + intercept` |
||||
|
||||
3. Add the fitted line to the plot. the plot should look like this: |
||||
|
||||
![alt text][q3] |
||||
|
||||
[q3]: images/w2_day1_ex2_q3.png "Scatter plot + fitted line" |
||||
|
||||
4. Predict on X |
||||
|
||||
5. Create a function that computes the Mean Squared Error (MSE) and compute the MSE on the data set. *The MSE is frequently used as well as other regression metrics that will be studied later this week.* |
||||
``` |
||||
def compute_mse(y_true, y_pred): |
||||
#TODO |
||||
return mse |
||||
``` |
||||
|
||||
Change the `noise` parameter of `make_regression` to 50 |
||||
|
||||
6. Repeat question 2, 4 and compute the MSE on the new data. |
||||
|
||||
https://scikit-learn.org/stable/modules/generated/sklearn.metrics.mean_squared_error.html |
||||
|
@ -0,0 +1,26 @@
|
||||
1. This question is validated if X_train, y_train, X_test, y_test match this output: |
||||
|
||||
```console |
||||
X_train: |
||||
[[ 1 2] |
||||
[ 3 4] |
||||
[ 5 6] |
||||
[ 7 8] |
||||
[ 9 10] |
||||
[11 12] |
||||
[13 14] |
||||
[15 16]] |
||||
|
||||
|
||||
y_train: |
||||
[1 2 3 4 5 6 7 8] |
||||
|
||||
|
||||
X_test: |
||||
[[17 18] |
||||
[19 20]] |
||||
|
||||
|
||||
y_test: |
||||
[ 9 10] |
||||
``` |
@ -0,0 +1,16 @@
|
||||
# Exercise 3: Train test split |
||||
|
||||
The goal of this exercise is to learn to split a data set. It is important to understand why we split the data in two sets. To put it in a nutshell: the Machine Learning model learns on the training data and evaluates on the data the model hasn't seen before: the testing data. |
||||
|
||||
This video gives a basic and nice explanation: https://www.youtube.com/watch?v=_vdMKioCXqQ |
||||
|
||||
This article explains the conditions to split the data and how to split it: https://machinelearningmastery.com/train-test-split-for-evaluating-machine-learning-algorithms/ |
||||
|
||||
```python |
||||
X = np.arange(1,21).reshape(10,-1) |
||||
y = np.arange(1,11) |
||||
``` |
||||
|
||||
1. Split the data using `train_test_split` with `shuffle=False`. The test set represents 20% of the total size of the data set. Print X_train, y_train, X_test, y_test. |
||||
|
||||
https://scikit-learn.org/stable/modules/generated/sklearn.model_selection.train_test_split.html |
@ -0,0 +1,60 @@
|
||||
1. This question is validated if the output of `y_train.values[:10]` and `y_test.values[:10]`are: |
||||
|
||||
```console |
||||
y_train.values[:10]: |
||||
[[202.] |
||||
[ 55.] |
||||
[202.] |
||||
[ 42.] |
||||
[214.] |
||||
[173.] |
||||
[118.] |
||||
[ 90.] |
||||
[129.] |
||||
[151.]] |
||||
|
||||
y_test.values[:10]: |
||||
[[ 71.] |
||||
[ 72.] |
||||
[235.] |
||||
[277.] |
||||
[109.] |
||||
[ 61.] |
||||
[109.] |
||||
[ 78.] |
||||
[ 66.] |
||||
[192.]] |
||||
``` |
||||
|
||||
2. This question is validated if the coefficients and the intercept are: |
||||
|
||||
```console |
||||
[('age', -60.40163046086952), |
||||
('sex', -226.08740652083418), |
||||
('bmi', 529.383623302316), |
||||
('bp', 259.96307686274605), |
||||
('s1', -859.121931974365), |
||||
('s2', 504.70960058378813), |
||||
('s3', 157.42034928335502), |
||||
('s4', 226.29533600601638), |
||||
('s5', 840.7938070846119), |
||||
('s6', 34.712225788519554), |
||||
('intercept', 152.05314895029233)] |
||||
``` |
||||
|
||||
3. This question is validated if the output of `predictions_on_test[:10]` is: |
||||
|
||||
```console |
||||
array([[111.74351759], |
||||
[ 98.41335251], |
||||
[168.36373195], |
||||
[255.05882934], |
||||
[168.43764643], |
||||
[117.60982186], |
||||
[198.86966323], |
||||
[126.28961941], |
||||
[117.73121787], |
||||
[224.83346984]]) |
||||
``` |
||||
|
||||
4. This question is validated if the mse on the **train set** is `2888.326888` and the mse on the **test set** is `2858.255153`. |
@ -0,0 +1,25 @@
|
||||
# Exercise 4 Forecast diabetes progression |
||||
|
||||
The goal of this exercise is to use Linear Regression to forecast the progression of diabetes. It will not always be precised, you should **ALWAYS** start doing an exploratory data analysis in order to have a good understanding of the data you model. As a reminder here an introduction to EDA: |
||||
|
||||
- https://towardsdatascience.com/exploratory-data-analysis-eda-a-practical-guide-and-template-for-structured-data-abfbf3ee3bd9 |
||||
|
||||
The data set used is described in https://scikit-learn.org/stable/modules/generated/sklearn.datasets.load_diabetes. |
||||
|
||||
```python |
||||
from sklearn.datasets import load_diabetes |
||||
diabetes = load_diabetes() |
||||
X, y = diabetes.data, diabetes.target |
||||
``` |
||||
|
||||
1. Using `train_test_split`, split the data set in a train set, and test set (20%). Use `random_state=43` for results reproducibility. |
||||
|
||||
2. Fit the Linear Regression on all the variables. Give the coefficients and the intercept of the Linear Regression. What is the the equation ? |
||||
|
||||
3. Predict on the test set. Predicting on the test set is like having new patients for who, as a physician, need to forecast the disease progression in one year given the 10 baseline variables. |
||||
|
||||
4. Compute the MSE on the train set and test set. Later this week we will learn about the R2 which will help us to evaluate the performance of this fitted Linear Regression. The MSE returns an arbitrary value depending on the range of error. |
||||
|
||||
**WARNING**: This will be explained later this week. But here, we are doing something "dangerous". As you may have read in the data documentation the data is scaled using the whole dataset whereas we should first scale the data on the training set and then use this scaling on the test set. This is a toy example, so let's ignore this detail for now. |
||||
|
||||
https://scikit-learn.org/stable/datasets/toy_dataset.html#diabetes-dataset |
@ -0,0 +1,46 @@
|
||||
1. This question is validated if the outputted plot looks like: |
||||
|
||||
![alt text][ex5q1] |
||||
|
||||
[ex5q1]: ../images/w2_day1_ex5_q1.png "Scatter plot " |
||||
|
||||
2. This question is validated if the output is: `11808.867339751561` |
||||
|
||||
3. This question is validated if `grid.shape` is `(640000,2)`. |
||||
|
||||
4. This question is validated if the 10 first values of losses are: |
||||
|
||||
```console |
||||
array([158315.41493175, 158001.96852692, 157689.02212209, 157376.57571726, |
||||
157064.62931244, 156753.18290761, 156442.23650278, 156131.79009795, |
||||
155821.84369312, 155512.39728829]) |
||||
``` |
||||
|
||||
5. This question is validated if the outputted plot looks like |
||||
|
||||
![alt text][ex5q5] |
||||
|
||||
[ex5q5]: ../images/w2_day1_ex5_q5.png "MSE" |
||||
|
||||
6. This question is validated if the point returned is |
||||
`array([42.5, 99. ])`. It means that `a= 42.5` and `b=99`. |
||||
|
||||
7. This question is validated if the coefficients returned are |
||||
|
||||
```console |
||||
Coefficients (a): 42.61943031121358 |
||||
Intercept (b): 99.18581814447936 |
||||
``` |
||||
|
||||
8. This question is validated if the outputted plot is |
||||
|
||||
![alt text][ex5q8] |
||||
|
||||
[ex5q8]: ../images/w2_day1_ex5_q8.png "MSE + Gradient descent" |
||||
|
||||
9. This question is validated if the coefficients and intercept returned are: |
||||
|
||||
```console |
||||
Coefficients: [42.61943029] |
||||
Intercept: 99.18581817296929 |
||||
``` |
After Width: | Height: | Size: 41 KiB |
After Width: | Height: | Size: 124 KiB |
After Width: | Height: | Size: 54 KiB |
After Width: | Height: | Size: 127 KiB |
@ -0,0 +1,124 @@
|
||||
|
||||
## Exercise 5 Gradient Descent - Optional |
||||
|
||||
The goal of this exercise is to understand how the Linear Regression algorithm finds the optimal coefficients. |
||||
|
||||
The goal is to fit a Linear Regression on a one dimensional features data **without using Scikit-learn**. Let's use the data set we generated for the exercise 2: |
||||
|
||||
```python |
||||
X, y, coef = make_regression(n_samples=100, |
||||
n_features=1, |
||||
n_informative=1, |
||||
noise=10, |
||||
coef=True, |
||||
random_state=0, |
||||
bias=100.0) |
||||
``` |
||||
|
||||
*Warning: The shape of X is not the same as the shape of y. You may need (for some questions) to reshape X using: `X.reshape(1,-1)[0]`.* |
||||
|
||||
1. Plot the data using matplotlib: |
||||
|
||||
![alt text][ex5q1] |
||||
|
||||
[ex5q1]: images/w2_day1_ex5_q1.png "Scatter plot " |
||||
|
||||
As a reminder, fitting a Linear Regression on this data means finding (a,b) that fits well the data points. |
||||
|
||||
- `y_pred = a*x +b` |
||||
|
||||
Mathematically, it means finding (a,b) that minimizes the MSE, which is the loss used in Linear Regression. If we consider 3 data points: |
||||
|
||||
- `Loss(a,b) = MSE(a,b) = 1/3 *((y_pred1 - y_true1)**2 + (y_pred2 - y_true2)**2) + (y_pred3 - y_true3)**2)` |
||||
|
||||
and we know: |
||||
|
||||
y_pred1 = a*x1 + b\ |
||||
y_pred2 = a*x2 + b\ |
||||
y_pred3 = a*x3 + b |
||||
|
||||
### Greedy approach |
||||
|
||||
2. Create a function `compute_mse`. Compute mse for `a = 1` and `b = 2`. |
||||
**Warning**: `X.shape` is `(100, 1)` and `y.shape` is `(100, )`. Make sure that `y_preds` and `y` have the same shape before to compute `y_preds-y`. |
||||
|
||||
```python |
||||
def compute_mse(coefs, X, y): |
||||
''' |
||||
coefs is a list that contains a and b: [a,b] |
||||
X is the features set |
||||
y is the target |
||||
|
||||
Returns a float which is the MSE |
||||
''' |
||||
|
||||
#TODO |
||||
|
||||
y_preds = |
||||
mse = |
||||
|
||||
return mse |
||||
``` |
||||
|
||||
3. Create a grid of **640000** points that combines a and b with. Check that the grid contains 640000 points. |
||||
|
||||
- a between -200 and 200, step= 0.5 |
||||
- b between -200 and 200, step= 0.5 |
||||
|
||||
This is how to compute the grid with the combination of a and b: |
||||
|
||||
```python |
||||
aa, bb = np.mgrid[-200:200:0.5, -200:200:0.5] |
||||
grid = np.c_[aa.ravel(), bb.ravel()] |
||||
``` |
||||
|
||||
4. Compute the MSE for all points in the grid. If possible, parallelize the computations. It may be needed to use `functools.partial` to parallelize a function with many parameters on a list. Put the result in a variable named `losses`. |
||||
|
||||
5. Use this chunk of code to plot the MSE in 2D: |
||||
|
||||
```python |
||||
aa, bb = np.mgrid[-200:200:.5, -200:200:.5] |
||||
grid = np.c_[aa.ravel(), bb.ravel()] |
||||
losses_reshaped = np.array(losses).reshape(aa.shape) |
||||
|
||||
f, ax = plt.subplots(figsize=(8, 6)) |
||||
contour = ax.contourf(aa, |
||||
bb, |
||||
losses_reshaped, |
||||
100, |
||||
cmap="RdBu", |
||||
vmin=0, |
||||
vmax=160000) |
||||
ax_c = f.colorbar(contour) |
||||
ax_c.set_label("MSE") |
||||
|
||||
ax.set(aspect="equal", |
||||
xlim=(-200, 200), |
||||
ylim=(-200, 200), |
||||
xlabel="$a$", |
||||
ylabel="$b$") |
||||
``` |
||||
|
||||
The expected output is: |
||||
|
||||
![alt text][ex5q5] |
||||
|
||||
[ex5q5]: images/w2_day1_ex5_q5.png "MSE " |
||||
|
||||
6. From the `losses` list, find the optimal value of a and b and plot the line in the scatter point of question 1. |
||||
|
||||
In this example we computed 160 000 times the MSE. It is frequent to deal with 50 features, which requires 51 parameters to fit the Linear Regression. If we try this approach with 50 features we would need to compute **5.07e+132** MSE. Even if we reduce the scope and try only 5 values per coefficients we would have to compute the MSE **4.4409e+35** times. This approach is not scalable and that is why is not used to find optimal coefficients for Linear Regression. |
||||
|
||||
### Gradient Descent |
||||
|
||||
In a nutshel, Gradient descent is an optimization algorithm used to minimize some function by iteratively moving in the direction of steepest descent as defined by the negative of the gradient. In machine learning, we use gradient descent to update the parameters (a and b) of our model. Parameters refer to the coefficients used in Linear Regression. Before to start implementing the questions, take the time to read the article. https://jairiidriss.medium.com/gradient-descent-algorithm-from-scratch-using-python-2b36c1548917. It explains the gradient descent and how to implement it. The "tricky" part is the computation of the derivative of the mse. You can admit the formulas of the derivatives to implement the gradient descent (`d_theta_0` and `d_theta_1` in the article). |
||||
|
||||
7. Implement the gradient descent to find optimal a and b with `learning rate = 0.1` and `nbr_iterations=100`. |
||||
|
||||
8. Save the a and b through the iterations in a two dimensional numpy array. Add them to the plot of the previous part and observe a and b that converge towards the minimum. The plot should look like this: |
||||
|
||||
![alt text][ex5q8] |
||||
|
||||
[ex5q8]: images/w2_day1_ex5_q8.png "MSE + Gradient descent" |
||||
|
||||
9. Use Linear Regression from Scikit-learn. Compare the results. |
@ -0,0 +1,54 @@
|
||||
# W2D01 Piscine AI - Data Science |
||||
|
||||
The goal of this day is to understand practical Linear regression and supervised learning. |
||||
|
||||
Author: |
||||
|
||||
# Table of Contents |
||||
|
||||
Historical part: |
||||
|
||||
# Introduction |
||||
|
||||
The word "regression" was introduced by Sir Francis Galton (a cousin of C. Darwin) when he |
||||
studied the size of individuals within a progeny. He was trying to understand why |
||||
large individuals in a population appeared to have smaller children, more |
||||
close to the average population size; hence the introduction of the term "regression". |
||||
|
||||
Today we will learn a basic algorithm used in **supervised learning** : **The Linear Regression**. We will be using **Scikit-learn** which is a machine learning library. It is designed to interoperate with the Python libraries NumPy and Pandas. |
||||
|
||||
We will also learn progressively the Machine Learning methodology for supervised learning - today we will focus on evaluating a machine learning model by splitting the data set in a train set and a test set. |
||||
|
||||
'0.22.1' |
||||
|
||||
## Rules |
||||
|
||||
## Ressources |
||||
|
||||
### To start with Scikit-learn |
||||
|
||||
- https://scikit-learn.org/stable/tutorial/basic/tutorial.html |
||||
|
||||
- https://jakevdp.github.io/PythonDataScienceHandbook/05.02-introducing-scikit-learn.html |
||||
|
||||
- https://scikit-learn.org/stable/modules/linear_model.html |
||||
|
||||
### Machine learning methodology and algorithms |
||||
|
||||
- This course provides a broad introduction to machine learning, datamining, and statistical pattern recognition. Andrew Ng is a star in the Machine Learning community. I recommend to spend some time during the projects to focus on some algorithms. However, Python is not the language used for the course. https://www.coursera.org/learn/machine-learning |
||||
|
||||
- https://docs.microsoft.com/en-us/azure/machine-learning/algorithm-cheat-sheet |
||||
|
||||
- https://scikit-learn.org/stable/tutorial/index.html |
||||
|
||||
### Linear Regression |
||||
|
||||
- https://towardsdatascience.com/laymans-introduction-to-linear-regression-8b334a3dab09 |
||||
|
||||
- https://towardsdatascience.com/linear-regression-the-actually-complete-introduction-67152323fcf2 |
||||
|
||||
### Train test split |
||||
|
||||
- https://machinelearningmastery.com/train-test-split-for-evaluating-machine-learning-algorithms/ |
||||
|
||||
- https://developers.google.com/machine-learning/crash-course/training-and-test-sets/video-lecture?hl=en |
@ -0,0 +1,24 @@
|
||||
1. This question is validated if the fitted logistic regression returns: |
||||
|
||||
```python |
||||
LogisticRegression(C=1.0, class_weight=None, dual=False, fit_intercept=True, |
||||
intercept_scaling=1, l1_ratio=None, max_iter=100, |
||||
multi_class='auto', n_jobs=None, penalty='l2', |
||||
random_state=0, solver='lbfgs', tol=0.0001, verbose=0, |
||||
warm_start=False) |
||||
``` |
||||
|
||||
2. This question is validated if the predicted class is `0`. |
||||
|
||||
3. This question is validated if the predicted probabilities are `[0.61450526 0.38549474]` |
||||
|
||||
4. This question is validated if the output is: |
||||
|
||||
```console |
||||
Coefficient: |
||||
[[0.81786797]] |
||||
Intercept: |
||||
[-0.87522391] |
||||
Score: |
||||
0.7142857142857143 |
||||
``` |
@ -0,0 +1,17 @@
|
||||
|
||||
# Exercise 1 Logistic regression in Scikit-learn |
||||
|
||||
The goal of this exercise is to learn to use Scikit-learn to classify data. |
||||
|
||||
```python |
||||
X = [[0],[0.1],[0.2], [1],[1.1],[1.2], [1.3]] |
||||
y = [0,0,0,1,1,1,0] |
||||
``` |
||||
|
||||
1. Fit a Logistic regression on X and y. |
||||
|
||||
2. Predict the class for `x_pred = [[0.5]]`. |
||||
|
||||
3. Predict the probabilities for `x_pred = [[0.5]]` using `predict_proba`. |
||||
|
||||
4. Print the coefficients (`coef_`), the intercept (`intercept_`) and the score of the logistic regression of X and y. |
@ -0,0 +1,5 @@
|
||||
1. This question is validated if the plot looks like this: |
||||
|
||||
![alt text][ex2q1] |
||||
|
||||
[ex2q1]: ../images/w2_day2_ex2_q1.png "Scatter plot" |
After Width: | Height: | Size: 53 KiB |
@ -0,0 +1,17 @@
|
||||
# Exercise 2 Sigmoid |
||||
|
||||
The goal of this exercise is to learn to compute and plot the sigmoid function. |
||||
|
||||
1. On the same plot, plot the sigmoid function and the custom sigmoids defined as: |
||||
|
||||
- `sigmoid1(x) = 1/(1+ exp(-(0.5*x + 3)))` |
||||
|
||||
- `sigmoid2(x) = 1/(1+ exp(-(5*x + 11)))` |
||||
|
||||
- Add a line representing the probability=0.5 |
||||
|
||||
The plot should look like this: |
||||
|
||||
![alt text][ex2q1] |
||||
|
||||
[ex2q1]: images/w2_day2_ex2_q1.png "Scatter plot" |
@ -0,0 +1,36 @@
|
||||
1. This question is validated if the outputted plot looks like this: |
||||
|
||||
![alt text][ex3q1] |
||||
|
||||
[ex3q1]: ../images/w2_day2_ex3_q1.png "Scatter plot" |
||||
|
||||
2. This question is validated if the coefficient and the intercept of the Logistic Regression are: |
||||
|
||||
```console |
||||
Intercept: [-0.98385574] |
||||
Coefficient: [[1.18866075]] |
||||
``` |
||||
|
||||
3. This question is validated if the plot looks like this: |
||||
|
||||
![alt text][ex3q2] |
||||
|
||||
[ex3q2]: ../images/w2_day2_ex3_q3.png "Scatter plot" |
||||
|
||||
4. This question is validated if `predict_probability` outputs the same probabilities as `predict_proba`. Note that the values have to match one of the class probabilities, not both. To do so, compare your output with: `clf.predict_proba(X)[:,1]`. The shape of the arrays is not important. |
||||
|
||||
5. This question is validated if `predict_class` outputs the same classes as `cfl.predict(X)`. The shape of the arrays is not important. |
||||
|
||||
6. This question is validated if the plot looks like this: |
||||
|
||||
![alt text][ex3q6] |
||||
|
||||
[ex3q6]: ../images/w2_day2_ex3_q5.png "Scatter plot + Logistic regression + predictions" |
||||
|
||||
As mentioned, it is not required to shift the class prediction to make the plot easier to understand. |
||||
|
||||
7. This question is validated if the plot looks like this: |
||||
|
||||
![alt text][ex3q7] |
||||
|
||||
[ex3q7]: ../images/w2_day2_ex3_q6.png "Logistic regression decision boundary" |
After Width: | Height: | Size: 30 KiB |
After Width: | Height: | Size: 47 KiB |
After Width: | Height: | Size: 50 KiB |
After Width: | Height: | Size: 121 KiB |
@ -0,0 +1,122 @@
|
||||
# Exercise 3 Decision boundary |
||||
|
||||
The goal of this exercise is to learn to fit a logistic regression on simple examples and to understand how the algorithm separated the data from the different classes. |
||||
|
||||
## 1 dimension |
||||
|
||||
First, we will start as usual with features data in 1 dimension. Use `make classification` from Scikit-learn to generate 100 data points: |
||||
|
||||
```python |
||||
X,y = make_classification( |
||||
n_samples=100, |
||||
n_features=1, |
||||
n_informative=1, |
||||
n_redundant=0, |
||||
n_repeated=0, |
||||
n_classes=2, |
||||
n_clusters_per_class=1, |
||||
weights=[0.5,0.5], |
||||
flip_y=0.15, |
||||
class_sep=2.0, |
||||
hypercube=True, |
||||
shift=1.0, |
||||
scale=1.0, |
||||
shuffle=True, |
||||
random_state=88 |
||||
) |
||||
``` |
||||
|
||||
*Warning: The shape of X is not the same as the shape of y. You may need (for some questions) to reshape X using: `X.reshape(1,-1)[0]`.* |
||||
|
||||
1. Plot the data using a scatter plot. The x-axis contains the feature and y-axis contains the target. |
||||
|
||||
The plot should look like this: |
||||
|
||||
![alt text][ex3q1] |
||||
|
||||
[ex3q3]: images/w2_day2_ex3_q3.png "Scatter plot" |
||||
|
||||
2. Fit a Logistic Regression on the generated data using scikit learn. Print the coefficients and the interception of the Logistic Regression. |
||||
|
||||
3. Add to the previous plot the fitted sigmoid and the 0.5 probability line. The plot should look like this: |
||||
|
||||
![alt text][ex3q3] |
||||
|
||||
[ex3q1]: images/w2_day2_ex3_q1.png "Scatter plot + Logistic regression" |
||||
|
||||
4. Create a function `predict_probability` that takes as input the data point and the coefficients and that returns the predicted probability. As a reminder, the probability is given by: `p(x) = 1/(1+ exp(-(coef*x + intercept)))`. Check you have the same results as the method `predict_proba` from Scikit-learn. |
||||
|
||||
```python |
||||
def predict_probability(coefs, X): |
||||
''' |
||||
coefs is a list that contains a and b: [coef, intercept] |
||||
X is the features set |
||||
|
||||
Returns probability of X |
||||
''' |
||||
#TODO |
||||
probabilities = |
||||
|
||||
return probabilities |
||||
``` |
||||
|
||||
5. Create a function `predict_class` that takes as input the data point and the coefficients and that returns the predicted class. Check you have the same results as the class method `predict` output on the same data. |
||||
|
||||
6. On the plot add the predicted class. The plot should look like this (the predicted class is shifted a bit to make the plot more understandable, but obviously the predicted class is 0 or 1, not 0.1 or 0.9) |
||||
The plot should look like this: |
||||
|
||||
![alt text][ex3q6] |
||||
|
||||
[ex3q6]: images/w2_day2_ex3_q5.png "Scatter plot + Logistic regression + predictions" |
||||
|
||||
## 2 dimensions |
||||
|
||||
Now, let us repeat this process on 2-dimensional data. The goal is to focus on the decision boundary and to understand how the Logistic Regression create a line that separates the data. The code to plot the decision boundary is provided, however it is important to understand the way it works. |
||||
|
||||
- Generate 500 data points using: |
||||
|
||||
```python |
||||
X, y = make_classification(n_features=2, |
||||
n_redundant=0, |
||||
n_samples=250, |
||||
n_classes=2, |
||||
n_clusters_per_class=1, |
||||
flip_y=0.05, |
||||
class_sep=3, |
||||
random_state=43) |
||||
``` |
||||
|
||||
7. Fit the Logistic Regression on X and y and use the code below to plot the fitted sigmoid on the data set. |
||||
|
||||
The plot should look like this: |
||||
|
||||
![alt text][ex3q7] |
||||
|
||||
[ex3q7]: images/w2_day2_ex3_q6.png "Logistic regression decision boundary" |
||||
|
||||
```python |
||||
xx, yy = np.mgrid[-5:5:.01, -5:5:.01] |
||||
grid = np.c_[xx.ravel(), yy.ravel()] |
||||
#if needed change the line below |
||||
probs = clf.predict_proba(grid)[:, 1].reshape(xx.shape) |
||||
|
||||
f, ax = plt.subplots(figsize=(8, 6)) |
||||
contour = ax.contourf(xx, yy, probs, 25, cmap="RdBu", |
||||
vmin=0, vmax=1) |
||||
ax_c = f.colorbar(contour) |
||||
ax_c.set_label("$P(y = 1)$") |
||||
ax_c.set_ticks([0, .25, .5, .75, 1]) |
||||
|
||||
ax.scatter(X[:,0], X[:, 1], c=y, s=50, |
||||
cmap="RdBu", vmin=-.2, vmax=1.2, |
||||
edgecolor="white", linewidth=1) |
||||
|
||||
ax.set(aspect="equal", |
||||
xlim=(-5, 5), ylim=(-5, 5), |
||||
xlabel="$X_1$", ylabel="$X_2$") |
||||
|
||||
``` |
||||
|
||||
The plot should look like this: |
||||
|
||||
- https://stackoverflow.com/questions/28256058/plotting-decision-boundary-of-logistic-regression |
@ -0,0 +1,31 @@
|
||||
|
||||
1. This question is validated if X_train, y_train, X_test, y_test match this output: |
||||
|
||||
```console |
||||
X_train: |
||||
[[ 1 2] |
||||
[ 3 4] |
||||
[ 5 6] |
||||
[ 7 8] |
||||
[ 9 10] |
||||
[11 12] |
||||
[13 14] |
||||
[15 16]] |
||||
|
||||
|
||||
y_train: |
||||
[0. 0. 0. 0. 0. 0. 0. 1.] |
||||
|
||||
|
||||
X_test: |
||||
[[17 18] |
||||
[19 20]] |
||||
|
||||
|
||||
y_test: |
||||
[1. 1.] |
||||
``` |
||||
|
||||
The proportion of class `1` is **0.125** in the train set and **1.** in the test set. |
||||
|
||||
2. This question is validated if the proportion of class `1` is **0.3** for both sets. |
@ -0,0 +1,20 @@
|
||||
|
||||
# Exercise 4: Train test split |
||||
|
||||
The goal of this exercise is to learn to split a classification data set. The idea is the same as splitting a regression data set but there's one important detail specific to the classification: the proportion of each class in the train set and test set. |
||||
|
||||
```python |
||||
X = np.arange(1,21).reshape(10,-1) |
||||
y = np.zeros(10) |
||||
y[7:] = 1 |
||||
``` |
||||
|
||||
1. Split the data using `train_test_split` with `shuffle=False`. The test set represents 20% of the total size of the data set. Print X_train, y_train, X_test, y_test. Compute the proportion of class `1` on the train set and test set. |
||||
|
||||
2. Having a train set with different properties than the test set is not recommended. The analogy of the exam (https://www.youtube.com/watch?v=_vdMKioCXqQ) helps to understand this point: if the questions you have at the exam are completely different from what you prepared for you are not evaluated on what you learn. The training set has to be representative of the data set. Now, split the data in a train set and test set, but keep the proportion of class `1` nearly constant. The parameter `shuffle` in theory works as it relies on a random sampling. The parameter `stratify` will always split the data and keep the same proportion of class `1` in the train set and test set. Using the parameter `stratify` split the data below and print the proportion of class `1` in the train set and train set. |
||||
|
||||
```python |
||||
X = np.arange(1,201).reshape(100,-1) |
||||
y = np.zeros(100) |
||||
y[70:] = 1 |
||||
``` |
@ -0,0 +1,56 @@
|
||||
1. This question is validated if the proportion of class `Benign` is 0.6552217453505007. It means that if you always predict `Benign` your accuracy would be 66%. |
||||
|
||||
2. This question is validated if the proportion of one of the classes is the approximately the same on the train and test set: ~0.65. In my case: |
||||
|
||||
- test: 0.6571428571428571 |
||||
- train: 0.6547406082289803 |
||||
|
||||
3. This question is validated if the output is: |
||||
|
||||
```console |
||||
# Train |
||||
Class prediction on train set: |
||||
[4 2 4 2 2 2 2 4 2 2] |
||||
|
||||
Probability prediction on train set: |
||||
[0.99600415 0.00908666 0.99992744 0.00528803 0.02097154 0.00582772 |
||||
0.03565076 0.99515326 0.00788281 0.01065484] |
||||
|
||||
Score on train set: |
||||
0.9695885509838998 |
||||
|
||||
#Test |
||||
|
||||
Class prediction on test set: |
||||
[2 2 2 4 2 4 2 2 2 4] |
||||
|
||||
Probability prediction on test set: |
||||
[0.01747203 0.22495309 0.00698756 0.54020801 0.0015289 0.99862249 |
||||
0.33607994 0.01227679 0.00438157 0.99972344] |
||||
|
||||
Score on test set: |
||||
0.9642857142857143 |
||||
|
||||
``` |
||||
|
||||
Only the 10 first predictions are outputted. The score is computed on all the data in the folds. |
||||
|
||||
For some reasons, you may have a different data splitting as mine. The requirement for this question is to have a score on the test set bigger than 92%. |
||||
|
||||
If the score is 1, congratulation you've leaked your first target. Drop the target from the X_train or X_test ;) ! |
||||
|
||||
4. This question is validated if the confusion matrix on the train set is similar to: |
||||
|
||||
```console |
||||
array([[357, 9], |
||||
[ 8, 185]]) |
||||
``` |
||||
|
||||
and if the confusion matrix on the test set is similar to: |
||||
|
||||
```console |
||||
array([[90, 2], |
||||
[ 3, 45]]) |
||||
``` |
||||
|
||||
As said, for some reasons, you may have slightly different results because of the data splitting. However, the values you have in the confusion matrix should be close to these results. |
@ -0,0 +1,23 @@
|
||||
# Exercise 5 Breast Cancer prediction |
||||
|
||||
The goal of this exercise is to use Logistic Regression |
||||
to predict breast cancer. It is always important to understand the data before training any Machine Learning algorithm. The data is described in **breast-cancer-wisconsin.names**. I suggest to add manually the column names in the DataFrame. |
||||
|
||||
Preliminary: |
||||
|
||||
- If needed, replace missing values with the median of the column. |
||||
|
||||
- Handle the column `Sample code number`. This column won't be used to train the model as it doesn't contain information on breast cancer. There are two solutions: drop it or set it as index. |
||||
|
||||
1. Print the proportion of class `Benign`. What would be the accuracy if the model always predicts `Benign`? |
||||
Later this week we will learn about other metrics as AUC that will help us to tackle high imbalanced data sets. |
||||
|
||||
2. Using train_test_split, split the data set in a train set and test set (20%). Both sets should have approximately the same proportion of class `Benign`. Use `random_state = 43`. |
||||
|
||||
3. Fit the logistic regression on the train set. Predict on the train set and test set. Compute the score on the train set and test set. 92-97% accuracy is expected on the test set. |
||||
|
||||
4. Compute the confusion matrix on both tests. Analyse the number of false negative and false positive. |
||||
|
||||
- https://scikit-learn.org/stable/modules/generated/sklearn.metrics.confusion_matrix.html |
||||
|
||||
- https://archive.ics.uci.edu/ml/machine-learning-databases/breast-cancer-wisconsin/ |
@ -0,0 +1,23 @@
|
||||
1. This question is validated if each classifier has as input a binary data as below: |
||||
|
||||
```python |
||||
def train(X_train, y_train): |
||||
clf = LogisticRegression() |
||||
clf1 = LogisticRegression() |
||||
clf2 = LogisticRegression() |
||||
|
||||
clf.fit(X_train, y_train == 0) |
||||
clf1.fit(X_train, y_train == 1) |
||||
clf2.fit(X_train, y_train == 2) |
||||
|
||||
return clf, clf1, clf2 |
||||
``` |
||||
|
||||
2. This question is validated if the predicted classes on the test set are: |
||||
|
||||
```console |
||||
array([0, 0, 2, 1, 2, 0, 2, 1, 1, 1, 0, 1, 2, 0, 1, 1, 0, 0, 2, 2, 0, 0, |
||||
0, 2, 2, 2, 0, 1, 0, 0]) |
||||
``` |
||||
|
||||
Even if I had this warning `ConvergenceWarning: lbfgs failed to converge (status=1):` I noticed that `LogisticRegression` returns the same output. |
@ -0,0 +1,59 @@
|
||||
|
||||
# Exercise 6 Multi-class (Optional) |
||||
|
||||
The goal of this exercise is to learn to train a classification algorithm on a multi-class labelled data. |
||||
Some algorithms as SVM or Logistic Regression do not natively support multi-class (more than 2 classes). There are some approaches that allow to use these algorithms on multi-class data. |
||||
Let's assume we work with 3 classes: A, B and C. |
||||
|
||||
- One-vs-Rest considers 3 binary classification problems: A vs B,C; B vs A,C and C vs A,B. If there are 10 classes, 10 binary classification problems would be fitted. |
||||
- One-vs-One considers 3 binary classification problems: A vs B, A vs C, B vs C. If there are 10 classes, 45 binary classification problems would be fitted. Given, the volume of data, this technique may not be scalable. |
||||
|
||||
More details: |
||||
|
||||
- https://machinelearningmastery.com/one-vs-rest-and-one-vs-one-for-multi-class-classification/ |
||||
|
||||
Let's implement the One-vs-Rest approach from `LogisticRegression`. |
||||
|
||||
Preliminary: |
||||
|
||||
- Import the Setosa data set from Scikit-learn |
||||
|
||||
```python |
||||
from sklearn.datasets import load_iris |
||||
iris = load_iris() |
||||
|
||||
X = pd.DataFrame(data=iris['data'], columns=iris.feature_names) |
||||
y = pd.DataFrame(data=iris['target'], columns=['target']) |
||||
``` |
||||
|
||||
- Using train_test_split, split the data set in a train set and test set (20%) with `shuffle=True` and `random_state=43`. |
||||
|
||||
1. Create a function that takes as input the data and returns three **trained** classifiers. |
||||
|
||||
- `clf0` takes as input a binary data set where the class 1 is `0` and class 0 is `1` and `2`. |
||||
- `clf1` takes as input a binary data set where the class 1 is `1` and class 0 is `0` and `2`. |
||||
- `clf2` takes as input a binary data set where the class 1 is `2` and class 0 is `0` and `1`. |
||||
|
||||
```python |
||||
def train(X_train,y_train): |
||||
#TODO |
||||
return clf0, clf1, clf2 |
||||
``` |
||||
|
||||
2. Create a function that takes as input the trained classifiers and the features set and that returns the predicted class. Use `predict_one_vs_all` to output the predicted classes on the test set. Compare the results with Logistic Regression algorithm from scikit learn used in One-vs-All mode. The results may change because the solver may not converge. Later this week, we will learn to preprocess the data to avoid convergence issues. |
||||
|
||||
- `clf0` outputs the probability to belong to the class 1 which is `0`. |
||||
- `clf1` outputs the probability to belong to the class 1 which is `1`. |
||||
- `clf2` outputs the probability to belong to the class 1 which is `2`. |
||||
|
||||
The predicted class is the one that gets the **highest probability** among the three models. |
||||
|
||||
```python |
||||
def predict_one_vs_all(X, clf0, clf1, clf2 ): |
||||
#TODO |
||||
return classes |
||||
``` |
||||
|
||||
- https://randerson112358.medium.com/python-logistic-regression-program-5e1b32f964db |
||||
|
||||
- https://towardsdatascience.com/logistic-regression-using-python-sklearn-numpy-mnist-handwriting-recognition-matplotlib-a6b31e2b166a |
@ -0,0 +1,44 @@
|
||||
# W2D02 Piscine AI - Data Science |
||||
|
||||
Classification |
||||
|
||||
# Table of Contents: |
||||
|
||||
# Introduction |
||||
|
||||
Classification |
||||
Today we will learn a different approach in Machine Learning: the classification which is a large domain in the field of statistics and machine learning. Generally, it can be broken down in two areas: |
||||
|
||||
- **Binary classification**, where we wish to group an outcome into one of two groups. |
||||
- **Multi-class classification**, where we wish to group an outcome into one of multiple (more than two) groups. |
||||
|
||||
You may wonder why the approach is different from regression and why we don't use regression and define a threshold from where the class would 1 else 0 - in binary classification. |
||||
The main reason is that the linear regression is sensitive to outliers, hence the treshold would vary depending on the outliers in the data. The article mentioned explains this reason with plots. To keep things simple, we can say that the output needed in classification is a probability to belong to one of the classes. So, by definition the value output by the classification model has to be between 0 and 1. The linear regression can't satisfy this constraint. |
||||
|
||||
In mathematics, there are functions with nice properties that take as input a real (-inf, inf) and output a value between 0 and 1, the most popular of them is the **sigmoid** - which is the inverse function of the logit, hence the name logistic regression. |
||||
|
||||
Let's take a small example to have a better understanding of the steps needed to perform a logistic regression on a binary data. Let's assume that we want to predict the gender given the people' size (height). |
||||
|
||||
Logistic regression steps: |
||||
|
||||
- Fit a sigmoid on the training data |
||||
- Compute sigmoid(size)=0.7 because the sigmoid returns values between 0 and 1 |
||||
- Return the class: 0.7 > 0.5 => class 1. Thus, the gender is male |
||||
|
||||
More details: |
||||
|
||||
- https://towardsdatascience.com/understanding-logistic-regression-9b02c2aec102 |
||||
|
||||
For the linear regression exercises, the loss (Mean Square Error - MSE) is minimized with an algorithm called **gradient descent**. In the classification, the loss MSE can't be used because the output of the model is 0 or 1 (for binary classification). |
||||
|
||||
The **logloss** or **cross entropy** is the loss used for classification. Similarly, it has some nice mathematical properties. The minimization of the **logloss** is not covered in the exercises. However, since it is used in most machine learning models for classification, I recommend to spend some time reading the related article. This article gives a nice example of how it works: |
||||
|
||||
- https://towardsdatascience.com/cross-entropy-for-classification-d98e7f974451 |
||||
|
||||
- https://medium.com/swlh/what-is-logistic-regression-62807de62efa |
||||
|
||||
## Historical |
||||
|
||||
## Rules |
||||
|
||||
## Ressources |