mirror of https://github.com/01-edu/Branch-AI.git
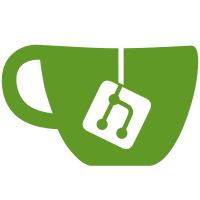
5 changed files with 119 additions and 79 deletions
@ -1,31 +1,47 @@
|
||||
# D03 Piscine AI - Data Science |
||||
# W1D03 Piscine AI - Data Science |
||||
|
||||
Author: |
||||
|
||||
# Introduction |
||||
## Visualizations |
||||
|
||||
While working on a dataset it is important to check the distribution of the data. Obviously, for most of humans it is difficult to visualize the data in more than 3 dimensions |
||||
|
||||
Viz is important to understand the data and to show results. We have already seen there are some basinc viz functionalities in Pandas. |
||||
Now we'll discover two of the most know viz libraries in Python: |
||||
"Viz" is important to understand the data and to show results. We'll discover three libraries to visualize data in Python. These are one of the most used visualisation "libraries" in Python: |
||||
|
||||
- Pandas viz |
||||
- Pandas visualization module |
||||
- Matplotlib |
||||
- Plotly |
||||
|
||||
Pandas viz is pratique: rapid plot, relies on Matplotlib. (check matplotlib doc sometimes not all params are detailed in pandas doc) |
||||
For more elaborate plots Matplotlib is necessary |
||||
The goal is to understand the basics of those libraries. You'll have time during the project to master one (or the three) of them. |
||||
You may wonder why using one library is not enough. The reason is simple: it depends on the usage. |
||||
For example if you want to check the data quickly you may want to use Pandas viz module or Matplotlib. |
||||
If you want to plot a custom and more elaborated plot I suggest to use Matplotlib or Plotly. |
||||
And, if you want to create a very nice and interactive plot I suggest to use Plotly. |
||||
|
||||
|
||||
## Exercises of the day |
||||
|
||||
- Exercise 1 Pandas plot 1 |
||||
- Exercise 2 Pandas plot 2 |
||||
- Exercise 3 Matplotlib 1 |
||||
- Exercise 4 Matplotlib 2 |
||||
- Exercise 5 Matplotlib subplots |
||||
- Exercise 6 Plotly 1 |
||||
- Exercise 7 Plotly Box plots |
||||
|
||||
And finaly Plotly is a interactive plot library.s |
||||
|
||||
## Rules |
||||
## Virtual Environment |
||||
- Python 3.x |
||||
- NumPy |
||||
- Pandas |
||||
- Matplotlib |
||||
- Plotly |
||||
- Jupyter or JupyterLab |
||||
|
||||
I suggest to use the most recent version of the packages. |
||||
|
||||
Always a title, legend, ...s |
||||
## Resources |
||||
|
||||
## Ressources |
||||
s |
||||
https://matplotlib.org/3.3.3/tutorials/index.html |
||||
https://towardsdatascience.com/matplotlib-tutorial-learn-basics-of-pythons-powerful-plotting-library-b5d1b8f67596 |
||||
- https://matplotlib.org/3.3.3/tutorials/index.html |
||||
- https://towardsdatascience.com/matplotlib-tutorial-learn-basics-of-pythons-powerful-plotting-library-b5d1b8f67596 |
||||
|
||||
https://github.com/rougier/matplotlib-tutorial |
||||
https://jakevdp.github.io/PythonDataScienceHandbook/05.13-kernel-density-estimation.html |
||||
- https://github.com/rougier/matplotlib-tutorial |
||||
- https://jakevdp.github.io/PythonDataScienceHandbook/05.13-kernel-density-estimation.html |
@ -1,33 +1,37 @@
|
||||
# D05 Piscine AI - Data Science |
||||
# W1D05 Piscine AI - Data Science |
||||
|
||||
The goal of this day is to understand practical usage of Pandas. |
||||
Today we will discover some important functionalities of Pandas. they will allow you to manipulate the data (DataFrame and Series) in order to clean, delete, add, merge and leverage more information. |
||||
## Time Series with Pandas |
||||
|
||||
In Data Science this is crucial, because without cleaned data there's no algorithms learning. |
||||
Time series data are data that are indexed by a sequence of dates or times. Today, you'll learn how to use methods built into Pandas to work with this index. You'll also learn for instance: |
||||
- to resample time series to change the frequency |
||||
- to calculate rolling and cumulative values for times series |
||||
- to build a backtest |
||||
|
||||
Author: |
||||
Time series a used A LOT in finance. You'll learn to evaluate financial strategies using Pandas. It is important to keep in mind that Python is vectorized. That's why some questions constraint you to not use a for loop ;-). |
||||
|
||||
# Table of Contents: |
||||
## Exercises of the day |
||||
|
||||
Historical part: |
||||
- Exercise 1 Series |
||||
- Exercise 2 Financial data |
||||
- Exercise 3 Multi asset returns |
||||
- Exercise 4 Backtest |
||||
|
||||
# Introduction |
||||
|
||||
Not only is the pandas library a central component of the data science toolkit but it is used in conjunction with other libraries in that collection. |
||||
## Virtual Environment |
||||
- Python 3.x |
||||
- NumPy |
||||
- Pandas |
||||
- Jupyter or JupyterLab |
||||
|
||||
Pandas is built on top of the NumPy package, meaning a lot of the structure of NumPy is used or replicated in Pandas. Data in pandas is often used to feed statistical analysis in SciPy, plotting functfunctionsions from Matplotlib, and machine learning algorithms in Scikit-learn. |
||||
*Version of Pandas I used to do the exercises: 1.0.1*. |
||||
I suggest to use the most recent one. |
||||
|
||||
## Historical |
||||
|
||||
## Rules |
||||
|
||||
... |
||||
|
||||
## Ressources |
||||
|
||||
Pandas website |
||||
## Resources |
||||
|
||||
- https://jakevdp.github.io/PythonDataScienceHandbook/ |
||||
|
||||
- https://pandas.pydata.org/Pandas_Cheat_Sheet.pdf |
||||
|
||||
- https://www.learndatasci.com/tutorials/python-pandas-tutorial-complete-introduction-for-beginners/ |
||||
|
||||
- https://towardsdatascience.com/different-ways-to-iterate-over-rows-in-a-pandas-dataframe-performance-comparison-dc0d5dcef8fe |
||||
|
Loading…
Reference in new issue