mirror of https://github.com/01-edu/Branch-AI.git
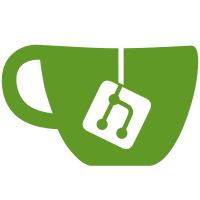
2 changed files with 109 additions and 14 deletions
@ -1,4 +1,76 @@
|
||||
https://github.com/XC-Li/Facial_Expression_Recognition/tree/master/Code/RAFDB |
||||
# Computer vision correction |
||||
|
||||
## CNN emotion classifier |
||||
|
||||
https://github.com/karansjc1/emotion-detection/tree/master/with%20flask |
||||
|
||||
### The first step is validated if: |
||||
- the model is trained only the training set |
||||
- the accuracy on the test set is higher than 70% |
||||
- the model learning curves should prove that the model is not overfitting |
||||
- the training has been stopped early enough to avoid the overfitting |
||||
- a screenshot shows the usage of the tensorboard |
||||
- the text document should explain why the architecture was chosen and what were the previous iterations |
||||
- predict.py runs without any error and returns: |
||||
|
||||
|
||||
```prompt |
||||
python predict.py |
||||
|
||||
Accuracy on test set: 72% |
||||
|
||||
``` |
||||
|
||||
|
||||
### The second step is validated if: |
||||
|
||||
|
||||
|
||||
- the preprocessing pipeline takes as input the webcam video stream and saves in a separate folder preprocessed* images |
||||
- preprocessing requirement*: |
||||
- sample at least one image per second |
||||
- the face is detected on the image |
||||
- the image is reshaped and centered (the face is at the center of the image) |
||||
- the algorithm that detects the face is imported via cv2 |
||||
- the image is converted to 48 x 48 grayscale pixels' image |
||||
- the trained model takes as input the preprocessed image and predicts the emotion with the associated probability |
||||
|
||||
- **predict_live_stream.py** runs without any error and returns: |
||||
|
||||
```prompt |
||||
python predict_live_stream.py |
||||
|
||||
Reading video stream ... |
||||
|
||||
Preprocessing ... |
||||
11:11:11s : Happy , 73% |
||||
|
||||
Preprocessing ... |
||||
11:11:12s : Happy , 93% |
||||
|
||||
Preprocessing ... |
||||
11:11:13s : Surprise , 71% |
||||
|
||||
Preprocessing ... |
||||
11:11:14s : Neutral , 82% |
||||
|
||||
... |
||||
|
||||
Preprocessing ... |
||||
11:13:29s : Happy , 63% |
||||
|
||||
``` |
||||
#### Hack the CNN - explanation: |
||||
|
||||
The neural network trains by updating its weights given the training error. If an image is misclassfied the neural network changes its weight to classify it correctly. The trick is to keep the neural network's weights unchanged and to modify the input pixels in order to force the neural network to predict the wanted class. |
||||
This part is validated if: |
||||
- an image from the database that gives more than 90% probability of Happy |
||||
- the neural network modifies the input pixels to predict Sad |
||||
- the modified image is SLIGHTLY changed. It means that you recognies very easily the original image. |
||||
- the predicted class of the modified image is Sad |
||||
|
||||
|
||||
|
||||
Here are three ressources that detail similar approaches: |
||||
- https://github.com/XC-Li/Facial_Expression_Recognition/tree/master/Code/RAFDB |
||||
- https://github.com/karansjc1/emotion-detection/tree/master/with%20flask |
||||
- https://www.kaggle.com/drbeanesp21/aliaj-final-facial-expression-recognition (simplified) |
||||
|
Loading…
Reference in new issue