mirror of https://github.com/01-edu/Branch-AI.git
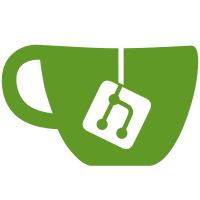
18 changed files with 3570 additions and 0 deletions
@ -0,0 +1,60 @@
|
||||
# Project01 - First Kaggle: Titanic - audit |
||||
|
||||
### Preliminary |
||||
|
||||
``` |
||||
project |
||||
│ README.md |
||||
│ environment.yml |
||||
│ username.txt |
||||
│ |
||||
└───data |
||||
│ │ train.csv |
||||
│ | test.csv |
||||
| | gender_submission.csv |
||||
│ |
||||
└───notebook |
||||
│ │ EDA.ipynb |
||||
| |
||||
|───scripts |
||||
│ |
||||
|
||||
``` |
||||
|
||||
###### Does the structure of the project is as below ? |
||||
|
||||
|
||||
###### Does the readme file give an introduction of the project, show the username, describe the feature engineering and show the best score the on the leaderboard ? |
||||
|
||||
###### Does the environment contain all libraries used and their versions that are necessary to run the code ? |
||||
|
||||
|
||||
|
||||
### Feature engineering |
||||
|
||||
|
||||
###### Does the notebook can be executed without any arror ? |
||||
|
||||
###### Does the notebook explain the feature engineering that contributed to improve the accuracy ? |
||||
|
||||
### Scripts |
||||
|
||||
###### Can you train the best model on the train data with feature engineering without any error ? |
||||
|
||||
###### Can you predict on the test set using the best model without any error ? |
||||
|
||||
###### Is the score you get **on the test set** with the best model is close to what is expected ? |
||||
|
||||
|
||||
### Final score |
||||
|
||||
###### Is the accuracy associated with the username in `username.txt` is higher than 79% ? The best submission score can be accessed from the user profile. |
||||
|
||||
|
||||
### Examples |
||||
|
||||
Here are two very good submissions explained and detailed: |
||||
|
||||
- https://www.kaggle.com/konstantinmasich/titanic-0-82-0-83 |
||||
- https://www.kaggle.com/sreevishnudamodaran/ultimate-eda-fe-neural-network-model-top-2 |
||||
|
@ -0,0 +1,116 @@
|
||||
# Your first Kaggle: Titanic |
||||
|
||||
## Introduction |
||||
|
||||
The goal of this **1 week** project is to get the highest possible score on a Data Science competition. More precisely you will have to predict who survived the Titanic crash. |
||||
|
||||
![alt text][titanic] |
||||
|
||||
[titanic]: titanic.jpg "Titanic" |
||||
|
||||
### Kaggle |
||||
|
||||
Kaggle is an online community of data scientists and machine learning practitioners. Kaggle allows users to find and publish data sets, explore and build models in a web-based data-science environment, work with other data scientists and machine learning engineers, and enter competitions to solve data science challenges. It’s a crowd-sourced platform to attract, nurture, train and challenge data scientists from all around the world to solve data science, machine learning and predictive analytics problems. |
||||
|
||||
### Titanic - Machine Learning from Disaster |
||||
|
||||
One of the first Kaggle competition I did was: Titanic - Machine Learning from Disaster. This is a not-to-be-missed Kaggle competition. |
||||
|
||||
You can see more [here](https://www.kaggle.com/c/titanic) |
||||
|
||||
The sinking of the Titanic is one of the most infamous shipwrecks in history. On April 15, 1912, during her maiden voyage, the widely considered “unsinkable” RMS Titanic sank after colliding with an iceberg. Unfortunately, there were not enough lifeboats for everyone onboard, resulting in the death of 1502 out of 2224 passengers and crew. |
||||
|
||||
While there was some element of luck involved in surviving, it seems some groups of people were more likely to survive than others. |
||||
|
||||
|
||||
In this challenge, you have to build a predictive model that answers the question: **“what sorts of people were more likely to survive?”** using passenger data (ie name, age, gender, socio-economic class, etc). **You will have to submit your prediction on Kaggle**. |
||||
|
||||
## Preliminary |
||||
|
||||
The way the Kaggle platform works is explained in the challenge overview page. If you need more details, I suggest this [resource](https://towardsdatascience.com/getting-started-with-kaggle-f9138b35ae18) that gives detailed explanations. |
||||
|
||||
- Create a username following this structure: username_01EDU_ location_MM_YYYY. Submit the description profile and push it on GitHub the first day of the week. Do not touch this file anymore. |
||||
|
||||
- It is possible to have different personal accounts merged in a team for one single competition. |
||||
|
||||
## Deliverables |
||||
|
||||
```console |
||||
project |
||||
│ README.md |
||||
│ environment.yml |
||||
│ username.txt |
||||
│ |
||||
└───data |
||||
│ │ train.csv |
||||
│ | test.csv |
||||
| | gender_submission.csv |
||||
│ |
||||
└───notebook |
||||
│ │ EDA.ipynb |
||||
| |
||||
|───scripts |
||||
│ |
||||
|
||||
``` |
||||
|
||||
- `README.md` introduction of the project, shows the username, describes the features engineering and the best score on the **leaderboard**. Note the score on the test set using the exact same pipeline that led to the best score on the leaderboard. |
||||
|
||||
- `environment.yml` contains all libraries required to run the code. |
||||
|
||||
- `username.txt` contains the username, the last modified date of the file **has to correspond to the first day of the project**. |
||||
|
||||
- `EDA.ipynb` contains the exploratory data analysis. This file should contain all steps of data analysis that contributed or not to improve the accuracy. It has to be commented so that the reviewer can understand the analysis and run it without any problem. |
||||
|
||||
- `scripts` contains python file(s) that perform(s) the feature engineering, the model's training and prediction on the test set. It could also be one single Jupyter Notebook. It has to be commented to help the reviewers understand the approach and run the code without any bugs. |
||||
- **Submit your predictions on the Kaggle's competition platform**. Check your ranking and score in the leaderboard. |
||||
|
||||
### Scores |
||||
|
||||
In order to validate the project you will have to score at least **79% accuracy on the Leaderboard**: |
||||
|
||||
- 79% accuracy is the minimum score to validate the project. |
||||
|
||||
Scores indication: |
||||
|
||||
- 79% difficult - minimum required |
||||
- 81% very difficult: smart feature engineering needed |
||||
- More than 83%: excellent that corresponds to the top 2% on Kaggle |
||||
- More than 85%: cheating |
||||
|
||||
#### Cheating |
||||
|
||||
It is impossible to get 100%. Who would have predicted that Rose wouldn't let [Jack on the door](https://www.insider.com/jack-and-rose-werent-on-a-door-in-titanic-2019-7) ? |
||||
|
||||
All people having 100% of accuracy on the Leaderboard cheated, there's no point to compare with them or to cheat. The Kaggle community estimates that having more than 85% is almost considered as cheated submissions as they are element of luck involved in the surviving. |
||||
|
||||
**You can't used external data sets than the ones provided in that competition.** |
||||
|
||||
## The key points |
||||
|
||||
- **Feature engineering**: |
||||
Put yourself in the shoes of an investigator trying to understand what happened exactly in that boat during the crash. Do not hesitate to watch the movie to try to find as many insights as possible. Without a smart the feature engineering there's no way to validate the project ;-) |
||||
|
||||
- The leaderboard evaluates on test data for which you don't have the labels. It means that there's no point to over fit the train set. Check the over fitting on the train set by dividing the data and by cross-validating the accuracy. |
||||
|
||||
## Advice |
||||
|
||||
Don't try to build the perfect model the first day. Iterate a lot and test your assumptions: |
||||
|
||||
Iteration 1: |
||||
|
||||
- Predict all passengers die |
||||
|
||||
Iteration 2 |
||||
|
||||
- Fit a logistic regression with a basic feature engineering |
||||
|
||||
Iteration 3: |
||||
|
||||
- Perform an EDA. Make assumptions and check them. Example: What if first class passengers survived more. Check the assumption through EDA and create relevant features to help the model capture the information. |
||||
|
||||
- Run a gridsearch |
||||
|
||||
Iteration 4: |
||||
|
||||
- Good luck ! |
After Width: | Height: | Size: 71 KiB |
diff.file_suppressed_line_too_long
diff.file_suppressed_line_too_long
@ -0,0 +1,109 @@
|
||||
# Project02 - NLP-enriched News Intelligence platform - audit |
||||
|
||||
### Preliminary |
||||
|
||||
``` |
||||
project |
||||
│ README.md |
||||
│ environment.yml |
||||
│ |
||||
└───data |
||||
│ │ topic_classification_data.csv |
||||
│ |
||||
└───results |
||||
│ │ topic_classifier.pkl |
||||
│ │ learning_curves.png |
||||
│ │ enhanced_news.csv |
||||
| |
||||
|───nlp_engine |
||||
│ |
||||
|
||||
``` |
||||
|
||||
###### Does the structure of the project is as below ? |
||||
|
||||
###### Does the readme file give an introduction of the project, show the username, describe the feature engineering and show the best score the on the leaderboard ? |
||||
|
||||
###### Does the environment contain all libraries used and their versions that are necessary to run the code ? |
||||
|
||||
|
||||
|
||||
|
||||
### Scrapper |
||||
|
||||
##### There are at least 300 news articles stored in the file system or the database. |
||||
##### Run the scrapper with `python scrapper_news.py` and fetch 3 documents. The scrapper is not expected to fetch 3 documents and stop by itself, you can stop it manually. It runs without any error and stores the 3 files as expected. |
||||
|
||||
### Topic classifier |
||||
|
||||
###### Are the learning curves provided ? |
||||
###### Do the learning curves prove the topics classifier is trained without correctly - without overfitting ? |
||||
###### Can you run the topic classfier model on the test set without any error ? |
||||
###### Does the topic classifier score an accuracy higher than 95% ? |
||||
|
||||
### Scandal detection |
||||
|
||||
###### Does the `README.md` explain the choice of embeddings and distance ? |
||||
|
||||
###### Does the DataFrame flag the top 10 articles with the highest likelihood of environmental scandal ? |
||||
|
||||
###### Is the distance or similarity saved in the DataFrame ? |
||||
|
||||
##### |
||||
### NLP engine output on 300 articles |
||||
|
||||
###### Does the DataFrame contain 300 different rows ? |
||||
###### Does the columns of the DataFrame are as expected ? |
||||
|
||||
``` |
||||
Date scrapped (date) |
||||
Title (str) |
||||
URL (str) |
||||
Body (str) |
||||
Org (str) |
||||
Topics (list str) |
||||
Sentiment (list float or float) |
||||
Scandal_distance (float) |
||||
Top_10 (bool) |
||||
|
||||
``` |
||||
|
||||
##### Analyse the DataFrame with 300 articles: relevance of the topics matched, relevance of the sentiment, relevance of the scandal detected and relevance of the companies matched. The algorithms are not 100% accurate so you should expect a few issues in the results. |
||||
|
||||
|
||||
### NLP engine on 3 articles |
||||
|
||||
###### Can you run `python nlp_enriched_news.py` without any error ? |
||||
|
||||
###### Does the output of the nlp engine correspond to the output below? |
||||
|
||||
```prompt |
||||
python nlp_enriched_news.py |
||||
|
||||
Enriching <URL>: |
||||
|
||||
Cleaning document ... (optional) |
||||
|
||||
---------- Detect entities ---------- |
||||
|
||||
Detected <X> companies which are <company_1> and <company_2> |
||||
|
||||
---------- Topic detection ---------- |
||||
|
||||
Text preprocessing ... |
||||
|
||||
The topic of the article is: <topic> |
||||
|
||||
---------- Sentiment analysis ---------- |
||||
|
||||
Text preprocessing ... (optional) |
||||
The title which is <title> is <sentiment> |
||||
The body of the article is <sentiment> |
||||
|
||||
---------- Scandal detection ---------- |
||||
|
||||
Computing embeddings and distance ... |
||||
|
||||
Environmental scandal detected for <entity> |
||||
``` |
||||
##### Analyse the output: relevance of the topic(s) matched, relevance of the sentiment, relevance of the scandal detected (if detected on the three articles) and relevance of the companie(s) matched. |
@ -0,0 +1,180 @@
|
||||
# NLP-enriched News Intelligence platform |
||||
|
||||
The goal of this project is to build an NLP-enriched News Intelligence platform. News analysis is a trending and important topic. The analysts get their information from the news and the amount of available information is limitless. Having a platform that helps to detect the relevant information is definitely valuable. |
||||
|
||||
|
||||
The platform connects to a news data source, detects the entities, detects the topic of the article, analyse the sentiment and ... |
||||
|
||||
## Scrapper |
||||
|
||||
News data source: |
||||
|
||||
- Find a news website that is easy to scrap. I could have chosen the website but the news' websites change their scraping policy frequently. |
||||
- Store it: |
||||
- File system per day: |
||||
- URL, date unique id |
||||
- headline |
||||
- body of the article |
||||
- SQL database (optional) |
||||
|
||||
from the last week otherwise the volume may be to high |
||||
|
||||
|
||||
## NLP engine |
||||
|
||||
In production architectures, the NLP engine delivers a live output based on the news that are delivered in a live stream data by the scrapper. However, it required advanced Python skills that are not a pre-requisite for the AI branch. |
||||
To simplify this step the scrapper and the NLP engine are used independently in the project. The scrapper fetches the news and store them in the data structure (either the file systeme or the SQL database) and then, the NLP engine runs on the stored data. |
||||
|
||||
Here how the NLP engine should process the news: |
||||
|
||||
### **1. Entities detection:** |
||||
|
||||
The goal is to detect all the entities in the document (headline and body). The type of entity we focus on is `ORG`. This corresponds to companies and organisations. This information should be stored. |
||||
|
||||
- Detect all companies using SpaCy NER on the body of the text. |
||||
|
||||
https://towardsdatascience.com/named-entity-recognition-with-nltk-and-spacy-8c4a7d88e7da |
||||
|
||||
|
||||
### **2. Topic detection:** |
||||
|
||||
The goal is to detect what the article is dealing with: Tech, Sport, Business, Entertainment or Politics. To do so, a labelled dataset is provided. From this dataset, build a classifier that learns to detect the right topic in the article. The trained model should be stored as `topic_classifier.pkl`. Make sure the model can be used easily (with the preprocessing pipeline built for instance) because the audit requires the auditor to test the model. |
||||
|
||||
Save the plot of learning curves (`learning_curves.png`) in `results` to prove that the model is trained correctly and not overfitted. |
||||
|
||||
- Learning constraints: **Score on test: > 95%** |
||||
|
||||
- **Optional**: If you want to train a news' topic classifier based on a more challenging dataset, you can use the following which based on 200k news headlines. https://www.kaggle.com/rmisra/news-category-dataset. |
||||
|
||||
|
||||
### **3. Sentiment analysis:** |
||||
|
||||
The goal is to detect the sentiment of the news articles. To do so, use a pre-trained sentiment model. I suggest to use: NLTK. |
||||
There are 3 reasons for which we use a pre-trained model: |
||||
|
||||
1. As a Data Scientist, you should learn to use a pre-trained model. There are so many models available and trained that sometimes you don't need to train one from scratch. |
||||
2. Labelled news data for sentiment analysis are very expensive. Companies as SESAMm provide this kind of services. |
||||
3. You already know how to train a sentiment analysis classifier ;-) |
||||
|
||||
### **4. Scandal detection ** |
||||
|
||||
The goal is to detect environmental disaster for the detected companies. Here is the methodology that should be used: |
||||
- Define keywords that correspond to environmental disaster that may be caused by companies: pollution, deforestation etc ... Here is an example of disaster we want to detect: https://en.wikipedia.org/wiki/MV_Erika. Pay attention to not use ambigous words that make sense in the context of an environmental disaster but also in another context. This would lead to detect a false positive natural disaster. |
||||
|
||||
- Compute the embeddings of the keywords. |
||||
|
||||
- Compute the distance between the embeddings of the keywords and all sentences that contain an entity. Explain in the `README.md` the embeddings chosen and why. Similarly explain the distance or similarity chosen and why. |
||||
|
||||
- Save the distance |
||||
|
||||
- Flag the top 10 articles. |
||||
|
||||
### 5. **Source analysis (optional)** |
||||
|
||||
The goal is to show insights about the news' source you scrapped. |
||||
This requires to scrap data on at least 5 days (a week ideally). Save the plots in the `results` folder. |
||||
|
||||
Here are examples of insights: |
||||
|
||||
- Per day: |
||||
|
||||
- Proportion of topics per day |
||||
- Number of articles |
||||
- Number of companies mentioned |
||||
- Sentiment per day |
||||
|
||||
- Per companies: |
||||
|
||||
- Companies mentioned the most |
||||
- Sentiment per companies |
||||
|
||||
|
||||
## Deliverables |
||||
|
||||
The structure of the project is: |
||||
|
||||
``` |
||||
project |
||||
│ README.md |
||||
│ environment.yml |
||||
│ |
||||
└───data |
||||
│ │ topic_classification_data.csv |
||||
│ |
||||
└───results |
||||
│ │ topic_classifier.pkl |
||||
│ │ learning_curves.png |
||||
│ │ enhanced_news.csv |
||||
| |
||||
|───nlp_engine |
||||
│ |
||||
|
||||
``` |
||||
|
||||
1. Run the scrapper until it fetches at least 300 articles |
||||
|
||||
``` |
||||
python scrapper_news.py |
||||
|
||||
1. scrapping <URL> |
||||
requesting ... |
||||
parsing ... |
||||
saved in <path> |
||||
|
||||
2. scrapping <URL> |
||||
requesting ... |
||||
parsing ... |
||||
saved in <path> |
||||
|
||||
``` |
||||
|
||||
2. Run on this 300 articles the NLP engine. |
||||
|
||||
Save a DataFrame: |
||||
|
||||
Date scrapped (date) |
||||
Title (str) |
||||
URL (str) |
||||
Body (str) |
||||
Org (str) |
||||
Topics (list str) |
||||
Sentiment (list float or float) |
||||
Scandal_distance (float) |
||||
Top_10 (bool) |
||||
|
||||
```prompt |
||||
python nlp_enriched_news.py |
||||
|
||||
Enriching <URL>: |
||||
|
||||
Cleaning document ... (optional) |
||||
|
||||
---------- Detect entities ---------- |
||||
|
||||
Detected <X> companies which are <company_1> and <company_2> |
||||
|
||||
---------- Topic detection ---------- |
||||
|
||||
Text preprocessing ... |
||||
|
||||
The topic of the article is: <topic> |
||||
|
||||
---------- Sentiment analysis ---------- |
||||
|
||||
Text preprocessing ... (optional) |
||||
The title which is <title> is <sentiment> |
||||
The body of the article is <sentiment> |
||||
|
||||
---------- Scandal detection ---------- |
||||
|
||||
Computing embeddings and distance ... |
||||
|
||||
Environmental scandal detected for <entity> |
||||
``` |
||||
|
||||
I strongly suggest to create a data structure (dictionary for example) to save all the intermediate result. Then, a boolean argument `cache` fetched the intermediate results when they are already computed. |
||||
|
||||
|
||||
Ressources: |
||||
|
||||
- https://www.youtube.com/watch?v=XVv6mJpFOb0 |
@ -0,0 +1,131 @@
|
||||
# Project03 - Computer vision - audit |
||||
|
||||
### Preliminary |
||||
|
||||
|
||||
``` |
||||
project |
||||
│ README.md |
||||
│ environment.yml |
||||
│ |
||||
└───data |
||||
│ │ train.csv |
||||
│ │ test.csv |
||||
│ │ xxx.csv |
||||
│ |
||||
└───results |
||||
│ │ |
||||
| |───model (free format) |
||||
│ │ │ my_own_model.pkl |
||||
│ │ │ my_own_model_architecture.txt |
||||
│ │ │ tensorboard.png |
||||
│ │ │ learning_curves.png |
||||
│ │ │ pre_trained_model.pkl (optional) |
||||
│ │ │ pre_trained_model_architecture.txt (optional) |
||||
│ │ |
||||
| |───hack_cnn (free format) |
||||
│ │ │ hacked_image.png (optional) |
||||
│ │ │ input_image.png |
||||
│ │ |
||||
| |───preprocessing_test |
||||
| | | input_video.mp4 (free format) |
||||
│ │ │ image0.png (free format) |
||||
│ │ │ image1.png |
||||
│ │ │ imagen.png |
||||
│ │ │ image20.png |
||||
| |
||||
|───scripts |
||||
│ │ train.py |
||||
│ │ predict.py |
||||
│ │ preprocess.py |
||||
│ │ predict_live_stream.py |
||||
│ │ hack_the_cnn.py |
||||
|
||||
``` |
||||
|
||||
###### Does the structure of the project is as below ? |
||||
|
||||
###### Does the readme file summurize how to run the code and explain the global approach ? |
||||
|
||||
###### Does the environment contain all libraries used and their versions that are necessary to run the code ? |
||||
|
||||
###### Do the text files explain the chosen architectures ? |
||||
|
||||
|
||||
|
||||
### CNN emotion classifier |
||||
|
||||
|
||||
###### Is the model trained only the training set ? |
||||
###### Is the accuracy on the test set is higher than 70% ? |
||||
###### Do the learning curves prove the model the model is not overfitting ? |
||||
###### Has the training been stopped early enough to avoid the overfitting ? |
||||
###### Does the screenshot show the usage of the tensorboard to monitor the training ? |
||||
###### Does the text document explain why the architecture was chosen and what were the previous iterations ? |
||||
###### Does the following command `python predict.py ` run without any error and returns an accuracy greater than 70% ? |
||||
|
||||
```prompt |
||||
python predict.py |
||||
|
||||
Accuracy on test set: 72% |
||||
|
||||
``` |
||||
|
||||
|
||||
### Face detection on the video stream |
||||
|
||||
|
||||
###### Does the preprocessing pipeline take as input the webcam video stream of minimum 20 sec and save in a separate folder at least 20 preprocessed* images ? |
||||
|
||||
###### Do all images contain a face ? |
||||
|
||||
###### Are all images reshaped and centered on the face ? |
||||
|
||||
###### Is the algorithm that detects the face imported via cv2 ? |
||||
|
||||
###### Is the image converted to 48 x 48 grayscale pixels' image |
||||
|
||||
###### If there's an issue related to the webcam, does the code takes as input a video recorded video stream ? |
||||
|
||||
###### Does the following command `predict_live_stream.py` run without any error and return the following ? |
||||
|
||||
```prompt |
||||
python predict_live_stream.py |
||||
|
||||
Reading video stream ... |
||||
|
||||
Preprocessing ... |
||||
11:11:11s : Happy , 73% |
||||
|
||||
Preprocessing ... |
||||
11:11:12s : Happy , 93% |
||||
|
||||
Preprocessing ... |
||||
11:11:13s : Surprise , 71% |
||||
|
||||
Preprocessing ... |
||||
11:11:14s : Neutral , 82% |
||||
|
||||
... |
||||
|
||||
Preprocessing ... |
||||
11:13:29s : Happy , 63% |
||||
|
||||
``` |
||||
|
||||
|
||||
### Hack the CNN - guidelines: |
||||
|
||||
The neural network trains by updating its weights given the training error. If an image is misclassfied the neural network changes its weight to classify it correctly. The trick is to keep the neural network's weights unchanged and to modify the input pixels in order to force the neural network to predict the wanted class. |
||||
This part is validated if: |
||||
|
||||
##### Choose an image from the database that gives more than 90% probability of `Happy` |
||||
###### Does the neural network modifies the input pixels to predict Sad ? |
||||
###### Can you recognize easily the chosen image ? The modified image is SLIGHTLY changed. It means that you recognies very easily the original image. |
||||
|
||||
|
||||
|
||||
Here are three ressources that detail similar approaches: |
||||
- https://github.com/XC-Li/Facial_Expression_Recognition/tree/master/Code/RAFDB |
||||
- https://github.com/karansjc1/emotion-detection/tree/master/with%20flask |
||||
- https://www.kaggle.com/drbeanesp21/aliaj-final-facial-expression-recognition (simplified) |
@ -0,0 +1,156 @@
|
||||
# Emotions detection with Deep Learning |
||||
Cameras are everywhere. Videos and images have become one of the most interesting data sets for artificial intelligence. |
||||
Image processing is a quite board research area, not just filtering, compression, and enhancement. Besides, we are even interested in the question, “what is in images?”, i.e., content analysis of visual inputs, which is part of the main task of computer vision. The study of computer vision could make possible such tasks as 3D reconstruction of scenes, motion capturing, and object recognition, which are crucial for even higher-level intelligence such as |
||||
image and video understanding, and motion understanding. |
||||
For this 2 months project we will focus on two tasks: |
||||
|
||||
- emotion classfication |
||||
- face tracking |
||||
|
||||
With the computing power exponentially increasing the computer vision field has been developping exponentially. This is a key element because the computer power allows to use more easily a type of neural networks very powerful on images: CNN's (Convolutional Neural Networks). Before the CNN's were democratized, the algorithms used relied a lot on human analysis to extract features which obviously time consuming and not reliable. If you're interested in the "old school methodology" this article explains it: towardsdatascience.com/classifying-facial-emotions-via-machine-learning-5aac111932d3. |
||||
The history behind this field is fascinating ! Here is a short summary of its history https://kapernikov.com/basic-introduction-to-computer-vision/ |
||||
|
||||
## Project goal and suggested timeline |
||||
|
||||
The goal of the project is to implement a **system that detects the emotion on a face from a webcam video stream**. To achieve this exciting task you'll have to understand how to: |
||||
|
||||
- deal with images in Python |
||||
- detect a face in an image |
||||
- train a CNN to detect the emotion on a face |
||||
|
||||
That is why I suggest to start the project with a preliminary step. The goal of this step is to understand how CNNs work and how to classify images. This preliminary step should take approximately **two weeks**. |
||||
|
||||
Then starts the emotion detection in a webcam video stream step that will last until the end of the project ! |
||||
|
||||
The two steps are detailed below. |
||||
|
||||
|
||||
## Preliminary: |
||||
|
||||
- Take this lesson. This course is a reference for many reasons and one of them is the creator: **Andrew Ng**. He explains the basics of CNNs but also some more advanced topics as transfer learning, siamese networks etc ... I suggest to focus on Week 1 and 2 and to spend less time on Week 3 and 4. Don't worry the time scoping of such MOOCs are conservative ;-). Here is the link: https://www.coursera.org/learn/convolutional-neural-networks . You can attend the lessons for free ! |
||||
|
||||
- Participate to this challenge: https://www.kaggle.com/c/digit-recognizer/code . The MNIST dataset is a reference in computer vision. Researchers use it as a benchmark to compare their models. Start first with a logistic regression to understand how to handle images in Python. And then train your first CNN on this data set. |
||||
|
||||
|
||||
## Face emotions classification |
||||
|
||||
|
||||
Emotion detection is one of the most researched topics in the modern-day machine learning arena. The ability to accurately detect and identify an emotion opens up numerous doors for Advanced Human Computer Interaction. The aim of this project is to detect up to seven distinct facial emotions in real time. This project runs on top of a Convolutional Neural Network (CNN) that is built with the help of Keras whose backend is TensorFlow in Python. The facial emotions that can be detected and classified by this system are Happy, Sad, Angry, Surprise, Fear, Disgust and Neutral. |
||||
|
||||
|
||||
Your goal is to implement a program that takes as input a video stream that contains a person's face and that predicts the emotion of the person. |
||||
|
||||
**Step 1**: **Fit the emotion classifier** |
||||
|
||||
- Train a CNN on the dataset `train.csv`. Here is an example of architecture you can implement: https://www.quora.com/What-is-the-VGG-neural-network . **The CNN has to perform more than 70% on the test set**. You will see that the CNNs take a lot of time to train. You don't want to overfit the neural network. I strongly suggest to use early stopping, callbacks and to monitor the training using the tensorboard. |
||||
|
||||
You have to save the trained model in `my_own_model.pkl` and to explain the chosen architecture in `my_own_model_architecture.txt`. Use `model.summary())` to print the architecture. It is also expected that you explains the iterations and how you end up choosing your final architecture. Save a screenshot of the tensorboard while the model's training in `tensorboard.png` and save a plot with the learning curves showing the model training and stopping BEFORE the model starts overfitting in `learning_curves.png`. |
||||
|
||||
- Optional: Use a pre-trained CNN to improve the accuracy. You will find some huge CNN's architecture that perform well. The issue is that it is expensive to train them from scratch. You'll need a lot of GPUs, memory and time. **Pre-trained CNNs** solve partially this issue because they are already trained on a dataset and perform well on some use cases. However, building a CNN from scratch is required, as mentioned, this step is optional and doesn't replace the first one. Similarly, save the model and explain the chosen architecture. |
||||
|
||||
**Step 2**: **Classify emotions from a video stream** |
||||
|
||||
|
||||
- Use the video stream outputted by your computer's webcam and preprocess it to make it compatible with the CNN you trained. One of the preprocessing steps is: face detection. As you may have seen the training samples are imaged centered on a face. To do so, I suggest to use a pre-trained model to detect faces. OpenCV for image processing tasks where we identify a face from a live webcam feed which is then processed and fed into the trained neural network for emotion detection. The preprocessing pipeline will be corrected with a functional test in `preprocessing_test`: |
||||
- **Input**: Video stream of 20 sec with a face on it |
||||
- **Output**: 20 (or 21) images cropped and centered on the face with 48 x 48 grayscale pixels |
||||
|
||||
- Predict at least one emotion per second from the video stream. The minimum requirement is printing in the prompt the predicted emotion with its associated probability. If there's any problem related to the webcam use as input the a recorded video stream. |
||||
|
||||
For that step, I suggest again to use **OpenCV** as much as possible: |
||||
|
||||
- https://opencv-python-tutroals.readthedocs.io/en/latest/py_tutorials/py_gui/py_video_display/py_video_display.html |
||||
|
||||
|
||||
- Optional: **(very cool)** Hack the CNN. Take a picture for which the prediction of your CNN is **Happy**. Now, hack the CNN: using the same image **SLIGHTLY** modified make the CNN predict **Sad**. https://medium.com/@ageitgey/machine-learning-is-fun-part-8-how-to-intentionally-trick-neural-networks-b55da32b7196 |
||||
|
||||
|
||||
## Deliverable |
||||
|
||||
|
||||
``` |
||||
project |
||||
│ README.md |
||||
│ environment.yml |
||||
│ |
||||
└───data |
||||
│ │ train.csv |
||||
│ │ test.csv |
||||
│ │ xxx.csv |
||||
│ |
||||
└───results |
||||
│ │ |
||||
| |───model (free format) |
||||
│ │ │ my_own_model.pkl |
||||
│ │ │ my_own_model_architecture.txt |
||||
│ │ │ tensorboard.png |
||||
│ │ │ learning_curves.png |
||||
│ │ │ pre_trained_model.pkl (optional) |
||||
│ │ │ pre_trained_model_architecture.txt (optional) |
||||
│ │ |
||||
| |───hack_cnn (free format) |
||||
│ │ │ hacked_image.png (optional) |
||||
│ │ │ input_image.png |
||||
│ │ |
||||
| |───preprocessing_test |
||||
| | | input_video.mp4 (free format) |
||||
│ │ │ image0.png (free format) |
||||
│ │ │ image1.png |
||||
│ │ │ imagen.png |
||||
│ │ │ image20.png |
||||
| |
||||
|───scripts |
||||
│ │ train.py |
||||
│ │ predict.py |
||||
│ │ preprocess.py |
||||
│ │ predict_live_stream.py |
||||
│ │ hack_the_cnn.py |
||||
|
||||
``` |
||||
|
||||
- Run **predict.py** expected output: |
||||
|
||||
```prompt |
||||
python predict.py |
||||
|
||||
Accuracy on test set: 72% |
||||
|
||||
``` |
||||
- Run **predict_live_stream.py** expected output: |
||||
|
||||
```prompt |
||||
python predict_live_stream.py |
||||
|
||||
Reading video stream ... |
||||
|
||||
Preprocessing ... |
||||
11:11:11s : Happy , 73% |
||||
|
||||
Preprocessing ... |
||||
11:11:12s : Happy , 93% |
||||
|
||||
Preprocessing ... |
||||
11:11:13s : Surprise , 71% |
||||
|
||||
Preprocessing ... |
||||
11:11:14s : Neutral , 82% |
||||
|
||||
... |
||||
|
||||
Preprocessing ... |
||||
11:13:29s : Happy , 63% |
||||
|
||||
``` |
||||
|
||||
## Useful ressources: |
||||
|
||||
- https://machinelearningmastery.com/what-is-computer-vision/ |
||||
|
||||
- Use a pre-trained CNN: https://arxiv.org/pdf/1812.06387.pdf |
||||
|
||||
- Hack the CNN https://medium.com/@ageitgey/machine-learning-is-fun-part-8-how-to-intentionally-trick-neural-networks-b55da32b7196 |
||||
|
||||
- http://ice.dlut.edu.cn/valse2018/ppt/WeihongDeng_VALSE2018.pdf |
||||
|
||||
- https://arxiv.org/pdf/1812.06387.pdf |
||||
|
After Width: | Height: | Size: 61 KiB |
@ -0,0 +1,133 @@
|
||||
# Financial strategies on the SP500 |
||||
|
||||
This documents is the correction of the project 4. Some steps are detailed in W1D5E4. TODO: replace with quest name |
||||
|
||||
|
||||
``` |
||||
project |
||||
│ README.md |
||||
│ environment.yml |
||||
│ |
||||
└───data |
||||
│ │ sp500.csv |
||||
│ |
||||
└───results |
||||
│ │ |
||||
| |───cross-validation |
||||
│ │ │ ml_metrics_train.csv |
||||
│ │ │ metric_train.csv |
||||
│ │ │ top_10_feature_importance.csv |
||||
│ │ │ metric_train.png |
||||
│ │ |
||||
| |───selected model |
||||
│ │ │ selected_model.pkl |
||||
│ │ │ selected_model.txt |
||||
│ │ │ ml_signal.csv |
||||
│ │ |
||||
| |───strategy |
||||
| | | strategy.png |
||||
│ │ │ results.csv |
||||
│ │ │ report.md |
||||
| |
||||
|───scripts (free format) |
||||
│ │ features_engineering.py |
||||
│ │ gridsearch.py |
||||
│ │ model_selection.py |
||||
│ │ create_signal.py |
||||
│ │ strategy.py |
||||
|
||||
``` |
||||
|
||||
###### Does the structure of the project is as below ? |
||||
|
||||
###### Does the readme file summurize how to run the code and explain the global approach ? |
||||
|
||||
###### Does the environment contain all libraries used and their versions that are necessary to run the code ? |
||||
|
||||
###### Do the text files explain the chosen model methodology ? |
||||
|
||||
|
||||
## **Data processing and feature engineering** |
||||
|
||||
###### Is the data splitted in a train set and test set ? |
||||
###### Is the last day of the train set is D and the first day of the test set is D+n with n>0 ? Splitting without considering the time series structure is wrong. |
||||
|
||||
##### There is no leakage: unfortunately there's no autamated way to check if the dataset is leaked. This step is validated if the features of date d are built as follow: |
||||
|
||||
| Index | Features |Target | |
||||
|----------|:-------------: |------:| |
||||
| Day D-1 | Features until D-1 23:59pm | return(D, D+1) | |
||||
| Day D | Features until D 23:59pm | return(D+1, D+2) | |
||||
| Day D+1 | Features until D+1 23:59pm | return(D+2, D+3) | |
||||
|
||||
###### Have the features been grouped by ticker before to compute the features ? |
||||
|
||||
###### - Has the target been grouped by ticker before to compute the futur returns ? |
||||
|
||||
|
||||
## **Machine Learning pipeline** |
||||
|
||||
### Cross-Validation |
||||
|
||||
###### Does the CV contain at least 10 folds in total ? |
||||
###### Do all train folds have more than 2y history ? If you use time series split, checking that the first fold has more than 2y history is enough. |
||||
##### The last validation set of the train set doesn't overlap on the test set. |
||||
##### None of the folds contain data from the same day.The split should be done on the dates. |
||||
##### There's a plot showing your cross-validation. As usual, all plots should have named axis and a title.If you chose a Time Series Split the plot should look like this: |
||||
|
||||
|
||||
![alt text][timeseries] |
||||
|
||||
[timeseries]: ../Time_series_split.png "Time Series split" |
||||
|
||||
|
||||
### Model Selection |
||||
|
||||
##### The test set hasn't been used to train the model and select the model. |
||||
###### Is the selected model saved in the pkl file and described in a txt file ? |
||||
|
||||
### Selected model |
||||
|
||||
##### The ml metrics computed on the train set are agregated: sum or median. |
||||
###### Are the ml metrics saved in a csv file ? |
||||
###### Are the top 10 important features per fold are saved in `top_10_feature_importance.csv`? |
||||
###### Does `metric_train.png` show a plot similar to the one below ? |
||||
*Note that, this can be done also on the test set **IF** this hasn't helped to select the pipeline. * |
||||
|
||||
![alt text][barplot] |
||||
|
||||
[barplot]: ../metric_plot.png "Metric plot" |
||||
|
||||
### Machine learning signal |
||||
|
||||
##### **The pipeline shouldn't be trained once and predict on all data points !** As explained: The signal has to be generated with the chosen cross validation: train the model on the train set of the first fold, then predict on its validation set; train the model on the train set of the second fold, then predict on its validation set, etc ... Then, concatenate the predictions on the validation sets to build the machine learning signal. |
||||
|
||||
|
||||
## **Strategy backtesting** |
||||
|
||||
### Convert machine learning signal into a strategy |
||||
|
||||
##### The transformed machine learning signal (long only, long short, binary, ternary, stock picking, proportional to probability or custom ) is multiplied by the return between d+1 and d+2. As a reminder, the signal at date d predicts wether the return between d+1 and d+2 is increasing or deacreasing. Then, the PnL of date d could be associated with date d, d+1 or d+2. This is arbitrary and should impact the value of the PnL. |
||||
|
||||
##### You invest the same amount of money every day. One exception: if you invest 1$ per day per stock the amount invested every day may change depending on the strategy chosen. If you take into account the different values of capital invested every day in the calculation of the PnL, the step is still validated. |
||||
|
||||
### Metrics and plot |
||||
|
||||
###### Is the Pnl computed as: strategy * futur_return ? |
||||
###### Does the strategy give the amount invested at time t on asset i ? |
||||
|
||||
###### Does the plot `strategy.png` contains an x axis: date ? |
||||
###### Does the plot `strategy.png` contains a y axis1: PnL of the strategy at time t ? |
||||
###### Does the plot `strategy.png` contains a y axis2: PnL of the SP500 at time t ? |
||||
###### Does the plot `strategy.png` use the same scale for y axis1 and y axis2 ? |
||||
###### Does the plot `strategy.png` contains a vertical line that shows the separation between train set and test set ? |
||||
|
||||
### Report |
||||
|
||||
###### Does the report detail the features used ? |
||||
|
||||
###### Does the report detail the pipeline used (imputer, scaler, dimension reduction and model) ? |
||||
|
||||
###### Does the report detail the cross-validation used (length of train sets and validation sets and if possible the cross-validation plot) ? |
||||
|
||||
###### Does the report detail the strategy chosen (description, PnL plot and the strategy metrics on the train set and test set) ? |
After Width: | Height: | Size: 68 KiB |
After Width: | Height: | Size: 56 KiB |
@ -0,0 +1,214 @@
|
||||
# Financial strategies on the SP500 |
||||
|
||||
TODO: data delivery and choose train/test split date. |
||||
|
||||
In this project we will apply machine to finance. You are a Quant/Data Scientist and your goal is to create a financial strategy based on a signal outputted by a machine learning model that overperforms the [SP500](https://en.wikipedia.org/wiki/S%26P_500). |
||||
|
||||
|
||||
The Standard & Poors 500 Index is a collection of stocks intended to reflect the overall return characteristics of the stock market as a whole. The stocks that make up the S&P 500 are selected by market capitalization, liquidity, and industry. Companies to be included in the S&P are selected by the S&P 500 Index Committee, which consists of a group of analysts employed by Standard & Poor's. |
||||
The S&P 500 Index originally began in 1926 as the "composite index" comprised of only 90 stocks. According to historical records, the average annual return since its inception in 1926 through 2018 is approximately 10%–11%.The average annual return since adopting 500 stocks into the index in 1957 through 2018 is roughly 8%. |
||||
As a Quant Researcher, you may beat the SP500 one year or few years. The real challenge though is to beat the SP500 consistently over decades. That's what most hedge funds in the world are trying to do. |
||||
|
||||
|
||||
The project is divided in parts: |
||||
|
||||
- **Data processing and feature engineering**: Build a dataset: insightful features and the target |
||||
- **Machine Learning pipeline**: Train machine learning models on the dataset, select the best model and generate the machine learning signal. |
||||
- **Strategy backtesting**: Generate a strategy from the Machine Learning model output and backtest the strategy. As a reminder, the idea here is to see what would have performed the strategy if you would have invested. |
||||
|
||||
## Deliverables |
||||
|
||||
Do not forget to check the ressources of W1D5 and espcially W1D5E4. |
||||
TODO: replace by quest name and exercice number |
||||
|
||||
### Data processing and features engineering |
||||
- Split the data in train and test (TODO: choose the year - once the data is delivered) |
||||
- Your first priority is to build a dataset without leakage !!! NO LEAKAGE !!! |
||||
|
||||
**"No leakage" small guide:** |
||||
We assume it is day D and we want to take a position on the next h days on the next day. The position starts on day D+1 (included). To decide wether we take a short or long position the return between day D+1 and D+2 is computed and used as a target. Finally, as features on day contain information until day D 11:59pm, target need to be shifted. As a result, the final dataframe schema is: |
||||
|
||||
|
||||
| Index | Features |Target | |
||||
|----------|:-------------: |------:| |
||||
| Day D-1 | Features until D-1 23:59pm | return(D, D+1) | |
||||
| Day D | Features until D 23:59pm | return(D+1, D+2) | |
||||
| Day D+1 | Features until D+1 23:59pm | return(D+2, D+3) | |
||||
|
||||
**Note: This table is simplified, the index of your DataFrame is a multi-index with date and ticker.** |
||||
|
||||
- Features: |
||||
- Bollinger |
||||
- RSI |
||||
- MACD |
||||
**Note: you can use any library to compute these features, you don't need to implement all financial features from scratch.** |
||||
|
||||
- Target: |
||||
- On day D, the target is: **sign(return(D+1, D+2))** |
||||
|
||||
> Remark: The target used is the return computed on the price and not the price directly. There are statistical reasons for this choice - the price is not stationary. The consequence is that a machine learning model tends to overfit while training on not stationary data. |
||||
|
||||
### Machine learning pipeline |
||||
|
||||
- Cross-validation deliverables: |
||||
- Implements a cross validation with at least 10 folds. The train set has to be bigger than 2 years history. |
||||
- Two types of temporal cross-validations are required: |
||||
- Blocking (plot below) |
||||
- Time Series split (plot below) |
||||
- Make sure the last fold of the train set does not overlap on the test set. |
||||
- Make sure the folds do not contain data from the same day. The data should be split on the dates. |
||||
- Plot your cross validation as follow: |
||||
|
||||
![alt text][blocking] |
||||
|
||||
[blocking]: blocking_time_series_split.png 'Blocking Time Series split' |
||||
|
||||
![alt text][timeseries] |
||||
|
||||
[timeseries]: Time_series_split.png 'Time Series split' |
||||
|
||||
Once you'll have run the gridsearch on the cross validation (choose either Blocking or Time Series split), you'll select the best pipeline on the train set and save it as `selected_model.pkl` and `selected_model.txt` (pipeline hyper-parameters). |
||||
|
||||
**Note: You may observe that the selected model is not good after analyzing the ml metrics (ON THE TRAIN SET) and select another one. ** |
||||
|
||||
- ML metrics and feature importances on the selected pipeline on the train set only. |
||||
- DataFrame with a Machine learning metrics on train et validation sets on all folds of the train set. Suggested format: columns: ML metrics (AUC, Accuracy, LogLoss), rows: folds, train set and validation set (double index). Save it as `ml_metrics_train.csv` |
||||
- Plot. Choose the metric you want. Suggested: AUC Save it as `metric_train.png`. The plot below shows how the plot should look like. |
||||
- DataFrame with top 10 important features for each fold. Save it as `top_10_feature_importance.csv` |
||||
|
||||
![alt text][barplot] |
||||
|
||||
[barplot]: metric_plot.png 'Metric plot' |
||||
|
||||
- The signal has to be generated with the chosen cross validation: train the model on the train set of the first fold, then predict on its validation set; train the model on the train set of the second fold, then predict on its validation set, etc ... Then, concatenate the predictions on the validation sets to build the machine learning signal. **The pipeline shouldn't be trained once and predict on all data points !** |
||||
|
||||
**The output is a DataFrame or Series with a double index ordered with the probability the stock price for asset i increases between d+1 and d+2.** |
||||
|
||||
- (optional): [Train a RNN/LSTM](https://towardsdatascience.com/predicting-stock-price-with-lstm-13af86a74944). This a nice way to discover and learn about recurrent neural networks. But keep in mind that there are some new neural network architectures that seem to outperform recurrent neural networks: https://towardsdatascience.com/the-fall-of-rnn-lstm-2d1594c74ce0. |
||||
|
||||
|
||||
## Strategy backtesting |
||||
|
||||
- Backtesting module deliverables. The module takes as input a machine learning signal, convert it into a financial strategy. A financial strategy DataFrame gives the amount invested at time t on asset i. The module returns the following metrics on the train set and the test set. |
||||
- PnL plot: save it as `strategy.png` |
||||
- x axis: date |
||||
- y axis1: PnL of the strategy at time t |
||||
- y axis2: PnL of the SP500 at time t |
||||
- Use the same scale for y axis1 and y axis2 |
||||
- add a line that shows the separation between train set and test set |
||||
- Pnl |
||||
- Max drawdown. https://www.investopedia.com/terms/d/drawdown.asp |
||||
- (Optional): add other metrics as sharpe ratio, volatility, etc ... |
||||
- Create a markdown report that explains and save it as `report.md`: |
||||
- the features used |
||||
- the pipeline used |
||||
- imputer |
||||
- scaler |
||||
- dimension reduction |
||||
- model |
||||
- the cross-validation used |
||||
- length of train sets and validation sets |
||||
- cross-validation plot (optional) |
||||
- strategy chosen |
||||
- description |
||||
- PnL plot |
||||
- strategy metrics on the train set and test set |
||||
|
||||
### Example of strategies: |
||||
- Long only: |
||||
- Binary signal: |
||||
0: do nothing for one day on asset i |
||||
1: take a long position on asset i for 1 day |
||||
- Weights proportional to the machine learning signals |
||||
- invest x on asset i for on day |
||||
- Long and short: For those who search long short strategy on Google, don't get wrong, this has nothing to do with pair trading. |
||||
- Binary signal: |
||||
- -1: take a short position on asset i for 1 day |
||||
- 1: take a long position on asset i for 1 day |
||||
- Ternary signal: |
||||
- -1: take a short position on asset i for 1 day |
||||
- 0: do nothing for one day on asset i |
||||
- 1: take a long position on asset i for 1 day |
||||
|
||||
Notes: |
||||
|
||||
- Warning! When you don't invest on all stock as in the binary signal or the ternary signal, make sure that you are still investing 1$ per day! |
||||
|
||||
- In order to simplify the **short position** we consider that this is the opposite of a long position. Example: I take a short one AAPL stock and the price decreases by 20$ on one day. I earn 20$. |
||||
|
||||
- Stock picking: Take a long position on the k best assets (from the machine learning signal) and short the k worst assets regarding the machine learning signal. |
||||
|
||||
Here's an example on how to convert a machine learning signal into a financial strategy: |
||||
|
||||
- Input: |
||||
|
||||
| Date | Ticker|Machine Learning signal | |
||||
|--------|:----: |-----------:| |
||||
| Day D-1| AAPL | 0.55 | |
||||
| Day D-1| C | 0.36 | |
||||
| Day D | AAPL | 0.59 | |
||||
| Day D | C | 0.33 | |
||||
| Day D+1| AAPL | 0.61 | |
||||
| Day D+1| C | 0.33 | |
||||
|
||||
- Convert it into a binary long only strategy: |
||||
- Machine learning signal > 0.5 |
||||
|
||||
| Date | Ticker|Binary signal | |
||||
|--------|:----: |-----------:| |
||||
| Day D-1| AAPL | 1 | |
||||
| Day D-1| C | 0 | |
||||
| Day D | AAPL | 1 | |
||||
| Day D | C | 0 | |
||||
| Day D+1| AAPL | 1 | |
||||
| Day D+1| C | 0 | |
||||
|
||||
|
||||
!!! BE CAREFUL !!!THIS IS EXTREMELY IMPORTANT. |
||||
|
||||
- Multiply it with the associated return. |
||||
|
||||
Don't forget the meaning of the signal on day d: it gives the return between d+1 and d+2. You should multiply the binary signal of day by the return computed between d+1 and d+2. Otherwise it's wrong because you use your signal that gives you information on d+1 and d+2 on the past or present. The strategy is leaked ! |
||||
|
||||
**Assumption**: you have 1$ per day to invest in your strategy. |
||||
|
||||
|
||||
## Project repository structure: |
||||
|
||||
``` |
||||
project |
||||
│ README.md |
||||
│ environment.yml |
||||
│ |
||||
└───data |
||||
│ │ sp500.csv |
||||
│ |
||||
└───results |
||||
│ │ |
||||
| |───cross-validation |
||||
│ │ │ ml_metrics_train.csv |
||||
│ │ │ metric_train.csv |
||||
│ │ │ top_10_feature_importance.csv |
||||
│ │ │ metric_train.png |
||||
│ │ |
||||
| |───selected model |
||||
│ │ │ selected_model.pkl |
||||
│ │ │ selected_model.txt |
||||
│ │ │ ml_signal.csv |
||||
│ │ |
||||
| |───strategy |
||||
| | | strategy.png |
||||
│ │ │ results.csv |
||||
│ │ │ report.md |
||||
| |
||||
|───scripts (free format) |
||||
│ │ features_engineering.py |
||||
│ │ gridsearch.py |
||||
│ │ model_selection.py |
||||
│ │ create_signal.py |
||||
│ │ strategy.py |
||||
|
||||
``` |
||||
Note: `features_engineering.py` can be used in `gridsearch.py` |
||||
|
||||
|
@ -0,0 +1,86 @@
|
||||
# Credit scoring |
||||
|
||||
## Preliminary |
||||
|
||||
``` |
||||
project |
||||
│ README.md |
||||
│ environment.yml |
||||
│ |
||||
└───data |
||||
│ │ ... |
||||
│ |
||||
└───results |
||||
│ │ |
||||
| |───model (free format) |
||||
│ │ │ my_own_model.pkl |
||||
│ │ │ model_report.txt |
||||
│ │ |
||||
| |feature_engineering |
||||
│ │ │ EDA.ipynb |
||||
│ │ |
||||
| |───clients_outputs |
||||
| | | client1_correct_train.pdf (free format) |
||||
│ │ │ client2_wrong_train.pdf (free format) |
||||
│ │ │ client_test.pdf (free format) |
||||
│ │ |
||||
| |───dashboard (optional) |
||||
| | | dashboard.py (free format) |
||||
│ │ │ ... |
||||
| |
||||
|───scripts (free format) |
||||
│ │ train.py |
||||
│ │ predict.py |
||||
│ │ preprocess.py |
||||
``` |
||||
###### Does the structure of the project is as below ? |
||||
|
||||
###### Does the readme file introduce the project, summurize how to run the code and show the username ? |
||||
|
||||
###### Does the environment contain all libraries used and their versions that are necessary to run the code ? |
||||
|
||||
###### Does the `EDA.ipynb` explain in details the exploratory data analysis ? |
||||
|
||||
|
||||
## Machine learning model |
||||
|
||||
###### Is the model trained only the training set ? |
||||
###### Is the AUC on the test set is higher than 75% ? |
||||
###### Does the model learning curves prove that the model is not overfitting ? |
||||
###### Has the training been stopped early enough to avoid the overfitting ? |
||||
###### Does the text document `model_report.txt` describe the methodology used to train the machine learning model ? |
||||
###### Does `predict.py` run without any error and returns the following ? |
||||
|
||||
|
||||
```prompt |
||||
python predict.py |
||||
|
||||
AUC on test set: 0.76 |
||||
|
||||
``` |
||||
|
||||
This [article](https://medium.com/thecyphy/home-credit-default-risk-part-2-84b58c1ab9d5) gives a complete example of a good modelling approach: |
||||
|
||||
|
||||
## Model's interpretability |
||||
|
||||
### Feature importance: |
||||
|
||||
###### Are the importance of all features used by the model computed and showed in a visualisation ? |
||||
|
||||
###### Is the mapping between between the importance of the features and the features' name is correct ? You should be careful here to associate the right variables to the their feature importance. Sometimes, the preprocessing pipeline can remove some features during the features selection step for instance. |
||||
|
||||
|
||||
### Descriptive variables: |
||||
##### These are important to understand for example the age of the client. If the data could be scaled or modified in the preprocessing pipeline but the data visualised here should be "raw". This part is validated if the visualisations are computed for the 3 clients. |
||||
- visualisations that show at least 10 variables describing the client and its loan(s) |
||||
- visualisations that show the comparison between this client and other clients. |
||||
|
||||
##### SHAP values on the model are displayed through a summary plot that shows the important features and their impact on the target. This is optional if you have already computed the features importance. |
||||
|
||||
###### Do the 3 clients are selected as expected ? 2 clients from the train set (1 on which the model is correct and 1 on which the model's wrong) and 1 client from the test set. |
||||
|
||||
##### SHAP values on predictions are computed for the 3 clients. The force plot shows what variables contributes the most to the score. **Check that the score outputted by the force plot corresponds to the one outputted by the model.** |
||||
|
||||
|
||||
|
After Width: | Height: | Size: 358 KiB |
@ -0,0 +1,46 @@
|
||||
# Credit scoring data description |
||||
|
||||
This file describes the available data for the project. |
||||
|
||||
 |
||||
|
||||
## application_{train|test}.csv |
||||
|
||||
This is the main table, broken into two files for Train (with TARGET) and Test (without TARGET). |
||||
Static data for all applications. One row represents one loan in our data sample. |
||||
|
||||
## bureau.csv |
||||
|
||||
All client's previous credits provided by other financial institutions that were reported to Credit Bureau (for clients who have a loan in our sample). |
||||
For every loan in our sample, there are as many rows as number of credits the client had in Credit Bureau before the application date. |
||||
|
||||
## bureau_balance.csv |
||||
|
||||
Monthly balances of previous credits in Credit Bureau. |
||||
This table has one row for each month of history of every previous credit reported to Credit Bureau – i.e the table has (#loans in sample * # of relative previous credits * # of months where we have some history observable for the previous credits) rows. |
||||
|
||||
## POS_CASH_balance.csv |
||||
|
||||
Monthly balance snapshots of previous POS (point of sales) and cash loans that the applicant had with Home Credit. |
||||
This table has one row for each month of history of every previous credit in Home Credit (consumer credit and cash loans) related to loans in our sample – i.e. the table has (#loans in sample * # of relative previous credits * # of months in which we have some history observable for the previous credits) rows. |
||||
|
||||
## credit_card_balance.csv |
||||
|
||||
Monthly balance snapshots of previous credit cards that the applicant has with Home Credit. |
||||
This table has one row for each month of history of every previous credit in Home Credit (consumer credit and cash loans) related to loans in our sample – i.e. the table has (#loans in sample * # of relative previous credit cards * # of months where we have some history observable for the previous credit card) rows. |
||||
|
||||
## previous_application.csv |
||||
|
||||
All previous applications for Home Credit loans of clients who have loans in our sample. |
||||
There is one row for each previous application related to loans in our data sample. |
||||
|
||||
|
||||
## installments_payments.csv |
||||
|
||||
Repayment history for the previously disbursed credits in Home Credit related to the loans in our sample. |
||||
There is a) one row for every payment that was made plus b) one row each for missed payment. |
||||
One row is equivalent to one payment of one installment OR one installment corresponding to one payment of one previous Home Credit credit related to loans in our sample. |
||||
|
||||
## HomeCredit_columns_description.csv |
||||
|
||||
This file contains descriptions for the columns in the various data files. |
Loading…
Reference in new issue